Spatial Single Cell Analysis of Proteins in 2D Human Gastruloids Using Iterative Immunofluorescence
Emily Freeburne, Emily Freeburne, Seth Teague, Seth Teague, Hina Khan, Hina Khan, Bolin Li, Bolin Li, Siyuan Ding, Siyuan Ding, Bohan Chen, Bohan Chen, Adam Helms, Adam Helms, Idse Heemskerk, Idse Heemskerk
Abstract
During development, cell signaling instructs tissue patterning, the process by which initially identical cells give rise to spatially organized structures consisting of different cell types. How multiple signals combinatorially instruct fate in space and time remains poorly understood. Simultaneous measurement of signaling activity through multiple signaling pathways and of the cell fates they control is critical to addressing this problem. Here we describe an iterative immunofluorescence protocol and computational pipeline to interrogate pattern formation in a 2D model of human gastrulation with far greater multiplexing than is possible with standard immunofluorescence techniques. This protocol and computational pipeline together enable imaging followed by spatial and co-localization analysis of over 27 proteins in the same gastruloids. We demonstrate this by clustering single cell protein expression, using techniques familiar from scRNA-seq, and linking this to spatial position to calculate spatial distributions and cell signaling activity of different cell types. These methods are not limited to patterning in 2D gastruloids and can be easily extended to other contexts. In addition to the iterative immunofluorescence protocol and analysis pipeline, Support Protocols for 2D gastruloid differentiation and producing micropatterned multi-well slides are included. © 2023 The Authors. Current Protocols published by Wiley Periodicals LLC.
Basic Protocol 1 : Iterative immunofluorescence
Basic Protocol 2 : Computational analysis pipeline
Support Protocol 1 : Generating micropatterned multi-well slides
Support Protocol 2 : Differentiation of 2D gastruloids
INTRODUCTION
Understanding how cell signaling instructs differentiation and tissue patterning is a central aim of developmental biology. Yet, measurement of the relationship between cell signaling and cell fate in space is challenging. Traditional immunofluorescence (IF) can visualize how cells are organized but is limited to a small number of antibodies due to spectral overlap and cross-reactivity. Techniques are needed to simultaneously measure sufficient cell fate markers and signaling pathways to dissect complex heterogeneous systems. Here, we provide protocols for interrogating cell fate and cell signaling in space using multiplexed IF data. Figure 1 summarizes the protocols and expected outputs.
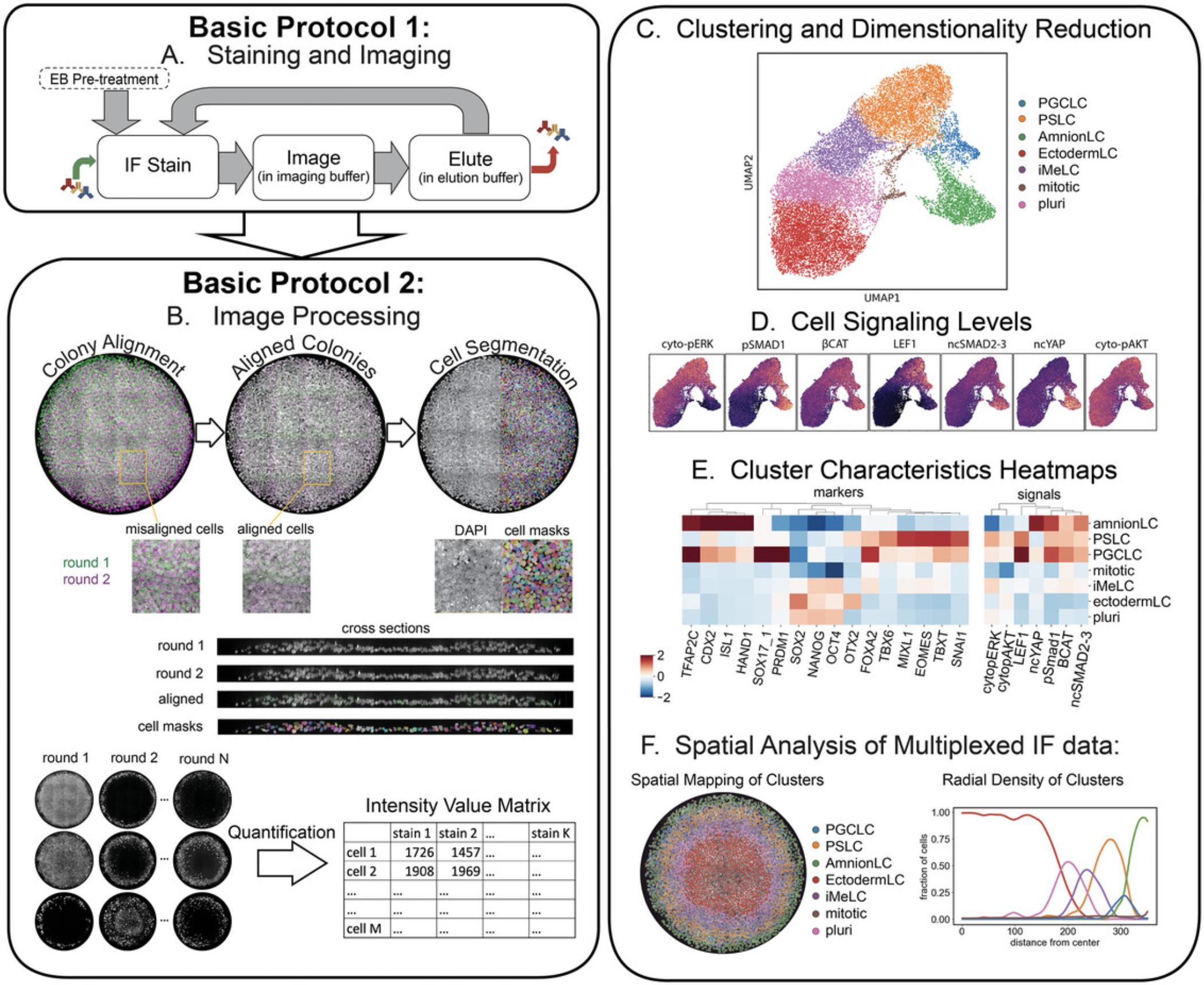
We apply these developed protocols to micropatterned human pluripotent stem cells (hPSCs) that, upon BMP4 treatment, undergo highly reproducible self-organized cell fate patterning and provide a powerful model for human gastrulation (Heemskerk, 2020). In this system, at least five different signaling pathways are known to play a role in patterning at least six different cell types, with most cell types requiring at least two marker genes to be identified (Chhabra et al., 2019; Jo et al., 2022; Kagiwada et al., 2021). As such, spatial single cell analysis of the relationship between signaling and fate is complex and requires highly multiplexed readouts. Importantly, this system is well-suited for quantitative image analysis because it is thin enough for high resolution microscopy. We optimized the 4i protocol (Iterative Indirect Immunofluorescence Imaging) by Gut et al. (2018) for use in this system, tested a large panel of antibodies relevant to early hPSC differentiation, and created a new image registration and analysis pipeline for single cell analysis in densely packed and multi-layered tissues.
Basic Protocol 1 describes the process of staining a sample using a modified 4i approach (Gut et al., 2018) and collecting the image data. This protocol involves (1) immunostaining, (2) image acquisition in a buffer that prevents photo-crosslinking of antibodies, (3) antibody removal using elution buffer, (4) microscopic imaging, and (5) repetitions of steps 1 to 4 to cover all desired proteins of interest. We included a crucial step of incubation in elution buffer between permeabilization and the first round of staining, which prevents tissue deformation between successive rounds due to this buffer, thereby enabling accurate image registration from successive rounds of IF imaging. The number of iterations of these steps is determined by the maximum number of antibodies raised in the same animal species desired for the particular experiment (e.g., 9 rounds if 9 different primary antibodies raised in mouse are planned). Basic Protocol 2 details how to extract a data matrix containing each cell's protein expression and signaling levels from the IF images. These data enable analyses of cell type heterogeneity, cell signaling, and spatial relationships between cells. Support Protocols 1 and 2 for generating micropatterned multi-well slides and culturing micropatterned gastruloids are also provided; however, the Basic Protocols are general enough to be used on most cell culture systems wherein layers of cells are attained that are thin enough for high resolution microscopy.
STRATEGIC PLANNING
Antibody Selection
We present a curated set of antibodies and a staining scheme in Tables 1 to 3 that were optimized for our application. In general, one may be interested in a different set of proteins and want to modify our staining scheme. Selecting which antibodies to use and determining their optimal order and concentration is an important consideration. We found that most, but not all, antibodies we tested are compatible with the buffers used in Basic Protocol 1. Some antibodies may yield non-specific background staining that is absent before treatment with elution buffer. Therefore, all antibodies should first be verified in a single round of staining before executing a multiplexed staining experiment. Originally, 4i used maleimide during the blocking step. However, we found that the quality of our pAKT stain was greatly reduced after the sample had been blocked with maleimide, while removing maleimide had no negative effects on the other stains. We therefore removed maleimide from our protocol.
Antigen | Manufacturer | Catalog number | Dilution |
---|---|---|---|
β-catenin | BD Biosciences | BDB610154 | 1/200 |
CDX2 | BioGenex | MU392A-5UC | 1/50 |
EOMES | R&D Systems | MAB6166 | 1/200 |
FOXA2 | R&D Systems | AF2400 | 1/500 |
GATA3 | SantaCruz Biotechnology | sc-268 | 1/50 |
HAND1 | R&D Systems | AF3168 | 1/200 |
ISL1 | DSHB | 39.4D5 | 1/200 |
LEF1 | Cell Signaling Tech | C12A5 | 1/100 |
MIXL1 | ABclonal | A17223 | 1/150 |
NANOG | R&D Systems | AF1997 | 1/100 |
OCT3/4 | BD Biosciences | 611202 | 1/400 |
OTX2 | R&D Systems | AF1979 | 1/150 |
pAKT (Ser473) | Cell Signaling Tech | 4060 | 1/200 |
pERK | Cell Signaling Tech | 9101S | 1/100 |
PRDM1 | SantaCruz Biotechnology | sc-47732 | 1/25 |
SMAD1 | Cell Signaling Tech | 6944T | 1/100 |
SMAD2 | Cell Signaling Tech | 5339T | 1/50 |
pSMAD1/5/9 | Cell Signaling Tech | 13820S | 1/50 |
SMAD2/3 | BD Biosciences | 610843 | 1/50 |
SNAI1 | R&D Systems | AF3639 | 1/100 |
SOX17 | R&D Systems | AF1924 | 1/1000 |
SOX2 | Cell Signaling Tech | 3579S | 1/200 |
TBX6 | R&D Systems | AF4744 | 1/100 |
TBXT | R&D Systems | AF2085 | 1/300 |
TFAP2C | SantaCruz Biotechnology | sc-12762 | 1/150 |
YAP | Cell Signaling Tech | 14074T | 1/100 |
Selecting an Antibody for Quality Control
To ensure that images are correctly registered to each other and that antigenicity is retained (i.e., that stains are reproducible between rounds), we advise performing quality control by repeating one of the first-round stains in a later round and calculating how the single cell intensities of this stain correlate between rounds. For the staining scheme in Table 3, we selected SOX17 for quality control and found 0.98 correlation between rounds 1 and 7 (see Critical Parameters). We selected SOX17 because of its scattered and relatively binary expression, for which registration errors reduce correlation between rounds more than for a more graded expression pattern. Although we found that stains are typically very reproducible between rounds, stains with less avidly binding antibodies and phospho-specific antibodies were less robust in later rounds. We therefore recommend staining with these antibodies in earlier rounds.
Imaging Parameters
Other antibodies may initially produce good quality stains yet display intensity decay in imaging buffer at room temperature over the course of many hours. Therefore, safeguards were implemented to minimize this risk of signal decay. To make sure intensity differences are minimal due to time in imaging buffer, we add imaging buffer to each well right before that well is imaged and limit imaging time to ∼1.5 hr per well. This is sufficient time to image 6 colonies at high resolution without noticeable fading (see Time Considerations).
Although longer exposures improve signal to noise, they also increase total imaging time. Moreover, both longer exposure and higher laser power can cause photo-crosslinking which interferes with elution. Therefore, we recommend keeping exposures and laser powers on the lower end. Generally, we used a maximum laser power of 5% for most antibodies but allowed up to 10% laser power and up to 200 ms for the poorest antibodies. See Table 1 for an example of antibody selection and imaging settings.
Antibody Dilution and Elution
Making sure that each antibody elutes completely is as important as checking whether the stain quality for each antibody is affected by this protocol. We have found that very bright stains can leave some residual signal after elution that would confound the signal of the following stain. Intuitively, this may be because removing 99.9% of a very high signal leaves enough to be detectable with exposures needed for a very dim stain, whereas after removing 99.9% of a very dim signal, it is no longer detectable with those settings. To prevent incomplete elution, we recommend diluting stronger antibodies to levels that require similar exposure as weaker antibodies to obtain the same signal to noise. For example, the standard dilution of our SOX17 antibody of 1:200 gave nuclear intensity values for positive nuclei on the order of 4000 a.u. using an exposure of 20 ms at 2% laser power in round 1. However, the LEF1 stain with a 1:100 dilution gave similar intensity values with 7% and 130 ms. Those same settings for SOX17 (7%, 130 ms) caused persistent signal detection after elution. Therefore, we increased the dilution of SOX17 to 1:1000 and correspondingly increased the imaging settings to 5% and 50 ms, which together attained similar signal intensity (i.e., ∼4000 a.u.) but no persistent detection after elution. Table 3 shows the imaging parameters used on our microscope for the corresponding primary antibody dilutions shown in Table 1.
NOTE : All protocols involving animals must be reviewed and approved by the appropriate Animal Care and Use Committee and must follow regulations for the care and use of laboratory animals. Appropriate informed consent is necessary for obtaining and use of human study material.
Basic Protocol 1: ITERATIVE IMMUNOFLUORESCENCE
This section describes how to execute iterative immunofluorescence stains using commonplace IF reagents and collect the image data with a confocal microscope. The result of this Basic Protocol 1 is a collection of images of the same cells over multiple rounds of staining that can be analyzed for protein expression, cell signaling, and spatial organization.
Materials
-
Cells (see Support Protocol 2)
-
Donkey block +/– Triton X-100 (see recipe)
-
Elution buffer (EB) (see recipe)
-
PBS, without Ca and Mg (Corning, cat. no. 21-040-CV)
-
Sodium azide (Sigma-Aldrich, cat. no. S8032-25G)
-
Primary antibody solution (see recipe)
-
PBST (see recipe)
-
Secondary antibody solution (see recipe)
-
Imaging buffer (IB) (see recipe)
-
Orbital shaker
-
Spinning disk confocal microscope
- Inverted microscope (Nikon Eclipse T i 2)
- Spinning disk (Yokogawa CSU-X1)
- Objective (Nikon PLAN APO 40×/1.25 SIL)
- Laser lines (405/488/561/640 nm)
- Software (NIS-Elements AR 5.41.02, build 1711)
-
Glass cleaner
-
Lens paper
-
JOBS protocol for NIS-Elements (see Data Availability Statement)
-
Stitching script (optional) (see Data Availability Statement)
-
Transfer script (optional) (see Data Availability Statement)
-
Immersion oil (Olympus SIL300CS-30CC)
-
14-ml conical tubes
-
1.5-ml Eppendorf tubes
-
Marker
-
Nitrile examination gloves, powder-free
-
Lab safety glasses
-
Lab coat
-
1-, 20-, 200- and 1000-µl pipette tips and pipettes
-
Serological pipettes
Pre-treat with elution buffer
1.Fix and store cells as described in Support Protocol 2.
2.Replace storage medium with 70 µl donkey block containing Triton X-100.
3.Incubate at room temperature for 2 hr.
4.Wash with 70 µl elution buffer (EB) 3 times for 10 min each.
5.Wash with PBS 3 times to rinse out EB.
Perform first stain
6.Incubate sample in donkey block with Triton X-100 for 30 min at room temperature.
7.Empty wells and add 70 µl primary antibody solution per well. Leave on the orbital shaker at 60 rpm for 3 hr at room temperature, protected from light.
8.Wash with 70 µl PBST 3 times for 15 min each.
9.Replace solution in wells with 70 µl secondary antibody solution.
10.Incubate sample protected from light on the orbital shaker for 1 hr at 60 rpm, room temperature.
11.Wash 2 times for 10 min each with PBST. Replace PBST with PBS.
12.Store sample protected from light until ready to image.
First round imaging
13.Clean the objective with glass cleaner and gently wiping the lens with lens paper. Power on Nikon microscope, open NIS software, and focus on the sample.
14.Click the “JOBS” tab, then click “Explorer.” Click “add” to add a new JOB protocol and import the JOBS protocol “230702_CP_JOBS.bin” from the supplementary files (see Data Availability Statement).
15.Lower the speed of the XY stage movement to two arrows as shown in Figure 2, this prevents losing the oil droplet from rapid stage movement.
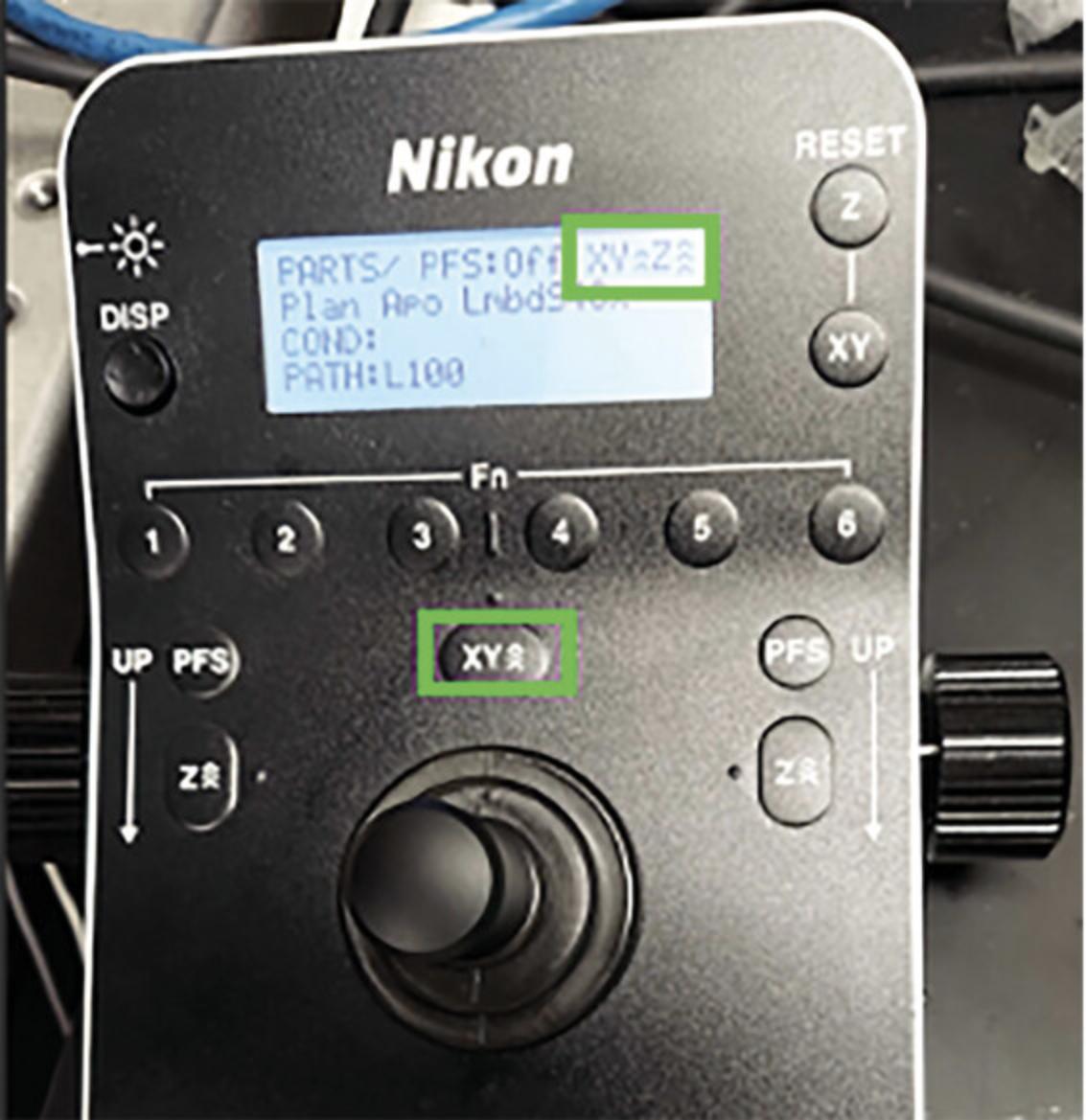
16.If multiple wells are to be imaged, determine the order in which to image them. Replace the medium with 80 µl imaging buffer (IB) in only that well right before it is ready to be imaged.
17.If imaging the first well, set the exposure and laser power settings for every channel with the guidelines discussed in the staining scheme section. Make note of the settings for later.
18.Center the view of the microscope on the center of each colony to be imaged and click “Add” to the ND Acquisition table (shown in Fig. 3). Pan around the colonies and set the z position to slightly lower than the lowest cell in the colony. Representative colonies should be selected.
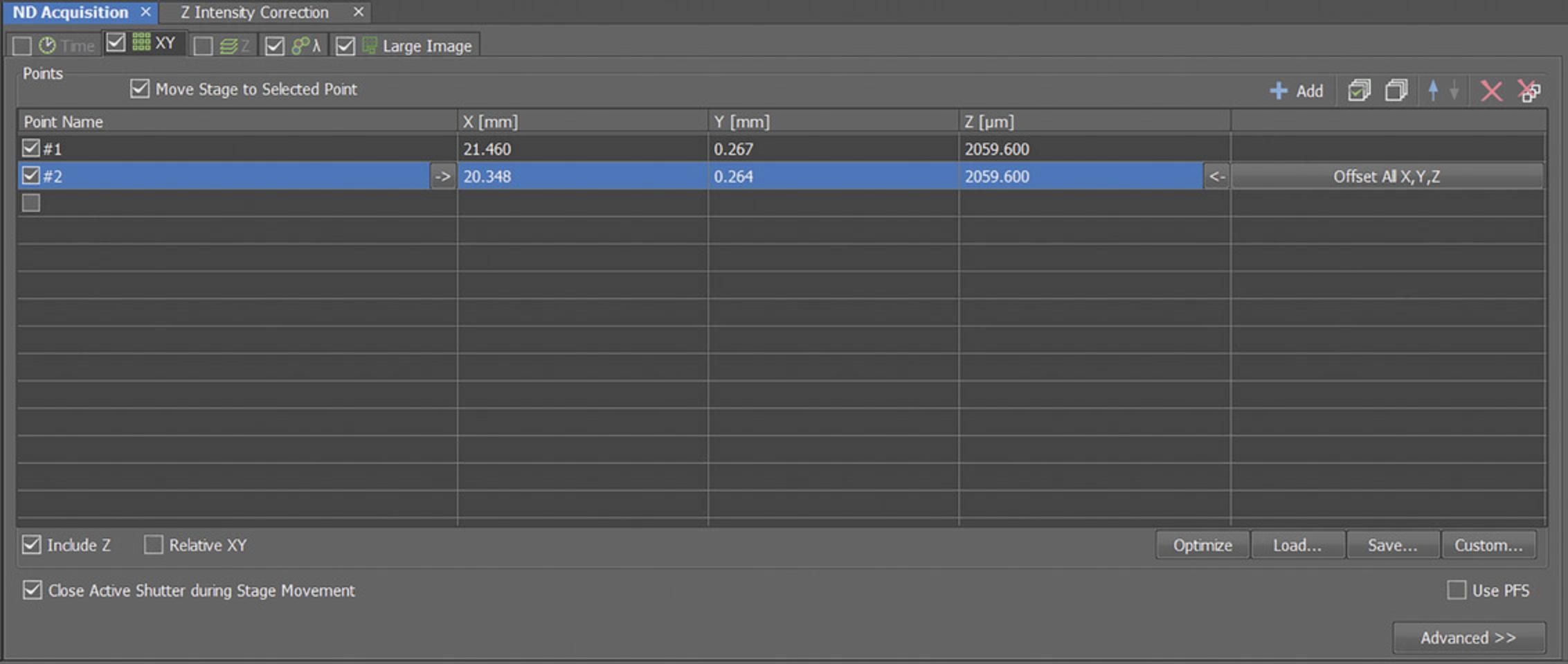
19.Save the XML file containing the list of positions to load during subsequent imaging.
20.Open the JOBS explorer and select the saved JOBS protocol from step 14.Click through the tabs on the left side of the window to ensure that all settings are correct and that only the correct well is selected in the “Select Wells” tab. Under the Storage tab, enter the desired file name and save location.
21.Click “Run” and you will be prompted to enter positions. Click “Load” and upload the XML file saved in step 19.
22.Next, the user will be prompted to set the z range for every colony.
-
Select “Symmetric Mode defined by range.”
-
Set the z range to 1 µm.
-
With the channel set to the DAPI channel (405 nm), click “Live” and lower the z position as necessary until it is set below the lowest cells, such that when imaged, all cells’ entire z range will be captured in the image.
-
Click “bottom” and then move up in z until just above the highest cell and click “top”.
-
Turn off “Live” as soon as possible to avoid unnecessary light exposure.
We set the number of z-slices on an individual basis per colony. Some variation is expected in the thickness of colonies. It is very important that cells are not cut off in z.
23.Click “next” after the last position is set. This will automatically begin the image acquisition.
24.Optional. Run the “stitchImages.m” script to monitor image acquisition in real time. This script periodically checks for new images, stitches them together, and saves these stitched images as TIFs for processing and jpgs for previewing.
-
Copy the stitchImages.m file into the destination folder of the unstitched nd2 images for every new round of imaging (recall that every round of imaging will be saved in its own subfolder within “data”).
-
Adjust the grid size (default: 6 × 4) and the waiting time in this script as necessary.
-
Then run the stitchImages script.
Stitching can also be run on a different computer by transferring the data to network storage during acquisition by first running the “Transfer.m” script on the computer connected to microscope. Open “Transfer.m” on MATLAB before or after image acquisition is started, and change “dir1” to the filepath of the images the microscope saves the new images into. Create a new folder in the network storage location with the same folder name. Change “dir2” to the filepath of the new folder created in the network storage. The advantage of stitching during imaging is that image quality can be monitored in real time. Issues such as oil droplet dislocation can be quickly corrected by restarting imaging if needed.
25.Restart from step 16 to continue imaging all stained wells until they are all imaged.
26.Once all imaging is complete, replace IB in all wells with 0.01% sodium azide in PBS for overnight storage.
Repeat staining until stain schedule is complete
27.Elute existing stain by washing with 70 µl EB, 3 times for 10 min each.
28.Wash with 70 µl PBS 3 times to rinse out EB.
29.Incubate wells in 70 µl donkey block without Triton X-100 for 30 min at room temperature.
30.Empty wells and add 70 µl primary antibody solution containing the appropriate antibody selections and leave on shaker at 60 rpm for 3 hr, protected from light.
31.Wash sample with 70 µl PBST 3 times for 15 min each.
32.Replace solution in wells with 70 µl secondary antibody solution.
33.Incubate sample for 1 hr at room temperature protected from light on shaker (60 rpm).
34.Wash with PBST 2 times for 10 min each.
35.Store sample protected from light until ready to image.
Imaging of later round staining
36.Load the XML file created from the first round of imaging (step 19), check the boxes corresponding to the correct colony positions within the target well.
37.Replace PBS with IB in only the well that is about to be imaged.
38.Check that the positions are correctly centered on the same colonies imaged during the first round of imaging, this includes checking that the z positioning is set beneath the lowest cell.
39.Start imaging from the JOBS protocol as previously described and optionally run step 24 again.
40.Store in 0.01% sodium azide in PBS overnight
41.Repeat from step 27 until the antibody schedule is complete and all images are collected.
Basic Protocol 2: COMPUTATIONAL ANALYSIS PIPELINE
This protocol extracts protein expression, cell signaling, and spatial information from images collected in Basic Protocol 1.This data can be used to identify and quantify heterogenous cell types, describe combinatorial cell signaling patterns in these cell types, and determine how these phenomena are governed in space. This process involves stitching image grids and aligning images of different rounds, then segmenting (creating masks) for every cell, and finally reading-out single cell expression levels from these masks (process summarized in Fig. 4). Our approach to cell segmentation uses the combination of two machine learning image segmentation tools, Ilastik (Berg et al., 2019) and Cellpose (Pachitariu & Stringer, 2022), to segment nuclei based on the DAPI stain from the first round of staining. Approximate cytoplasmic masks are then generated automatically by creating annuli around each nuclear mask (excluding neighboring nuclei). The key result of Basic Protocol 2 is a matrix of single cell intensity data for every stain that also includes spatial coordinates of the cell. This can then be used for further analysis of cell type heterogeneity, signaling, and spatial organization which we illustrate by creating UMAP plots, clustering fates, and making plots of spatial distribution of clusters as shown in Figure 1. While prior coding experience is not required to follow the protocol, we recommend some basic familiarity with MATLAB and Python languages.
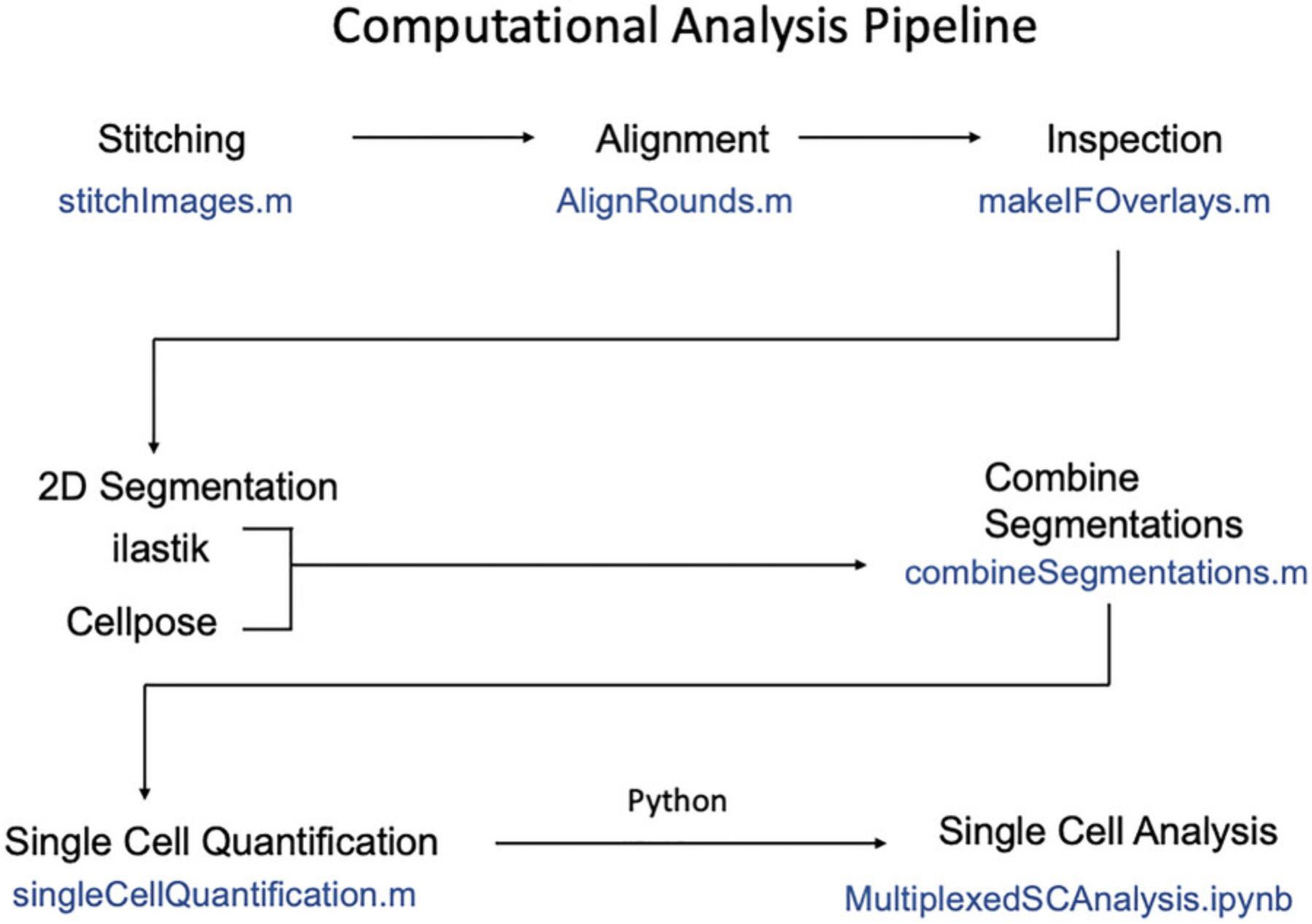
Necessary Resources
- MATLAB (2021a or newer, requires Imaging Processing Toolbox and Computer Vision Toolbox)
- Scripts for image analysis included as supplementary files (see Data Availability Statement) and at github.com/idse/6i
- Python 3.8.0
- Numerous Python packages defined in supplementary file Mplex_analysis.yml including Cellpose (see Data Availability Statement)
- Anaconda (see Internet Resources), or another tool to set up a Python environment
- Ilastik (1.3.3post3-OSX) (see Internet Resources)
Stitching and alignment
1.If not already performed in Basic Protocol 1 step 24, copy “stitchImages.m” into each folder containing unstitched nd2 files (i.e., each folder that corresponds to each round) and execute the scripts in each folder. Review the preview images for quality.
2.Align images for the same colonies across different rounds of staining by running “AlignRounds.m”. For your own experiment, change the names of the folders listed in the “dirs” variable in line 11 to match the names of all the folders in your data set.
3.Optional. Create RGB overlays of maximal intensity projection of any 3 stains from any round(s) by running “makeIFOverlays.m”. Select which stains to visualize by changing the channels variable in line 50. Note the first name in the list will be colored red, the second will be green, and the third will be blue. For your own experiment, change the name of the folders listed in the “dirs” variable in line 10 to match the names of all the folders of the rounds in your data set.
Cell segmentation
4.Create the Mplex_analysis environment.
For Mac computers
-
Open the terminal.
-
Navigate to the “code” folder from the supplementary files (see Data Availability Statement).
-
Enter the following into the terminal:conda env create -f Mplex_analysis.yml
For Windows computers
-
Open the anaconda prompt.
-
Navigate to the “code” folder from the supplementary files (see Data Availability Statement).
-
Create the separate cellpose environment:conda env create -f cellpose_environment.yml
5.Activate the Mplex_analysis environment.
For Mac computers
-
Enterconda activate Mplex_analysisto activate the environment, then enterjupyter labto launch JupyterLab.
For Windows computers
- b.Enter conda activate cellpose then enter jupyter lab to launch JupyterLab.
6.Copy ‘segmentation_cellpose.ipynb’ into the round 1 folder containing the stitched images and launch jupyter notebook. Run Cellpose cell segmentation from this script.
7.Open Ilastik and select “Pixel Classification” under “Create New Project.” Select a folder to save the ilastik project file into.
8.Click the “Add New” button under the empty table header, then click “Add separate Image(s)” and add representative images from the DAPI channel from the first round of staining as the training data. These will have filenames of the format “stitched_pxxxx_w0000_t0000.tif” where p indicates the colony (p0000 represents the first colony imaged), w the channel (w0000 being the DAPI channel), and t the time (which is always t0000 given that these are fixed samples).
9.Navigate to the Feature Selection tab on the left of the window, click Select Features and highlight all boxes in the Features window (shown in Fig. 5) then click ‘OK’.
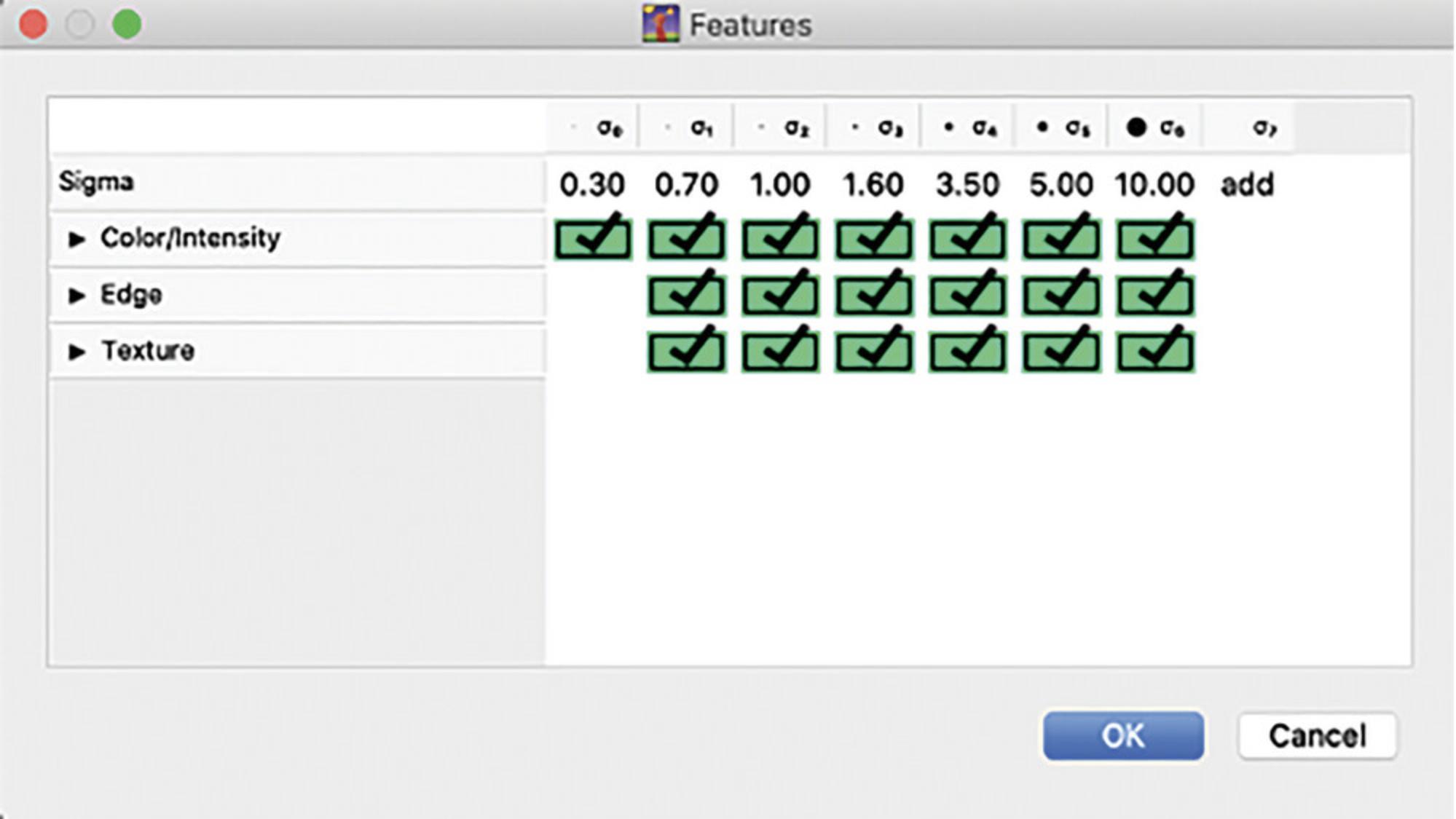
10.Click the Training tab and create labels for pixels that are cells versus background, we use “Label 1” as cell and “Label 2” as non-cell background.
11.Create masks by drawing over cell nuclei and background areas with the correct labels as illustrated in Figure 6.Scroll through different z slices by using the “time” scrollbar at the bottom right of the window (this is not “time” but z slices). Click “Live update” and then check “segmentation” under Group visibility to view how well the classifier is performing on all the pixels.
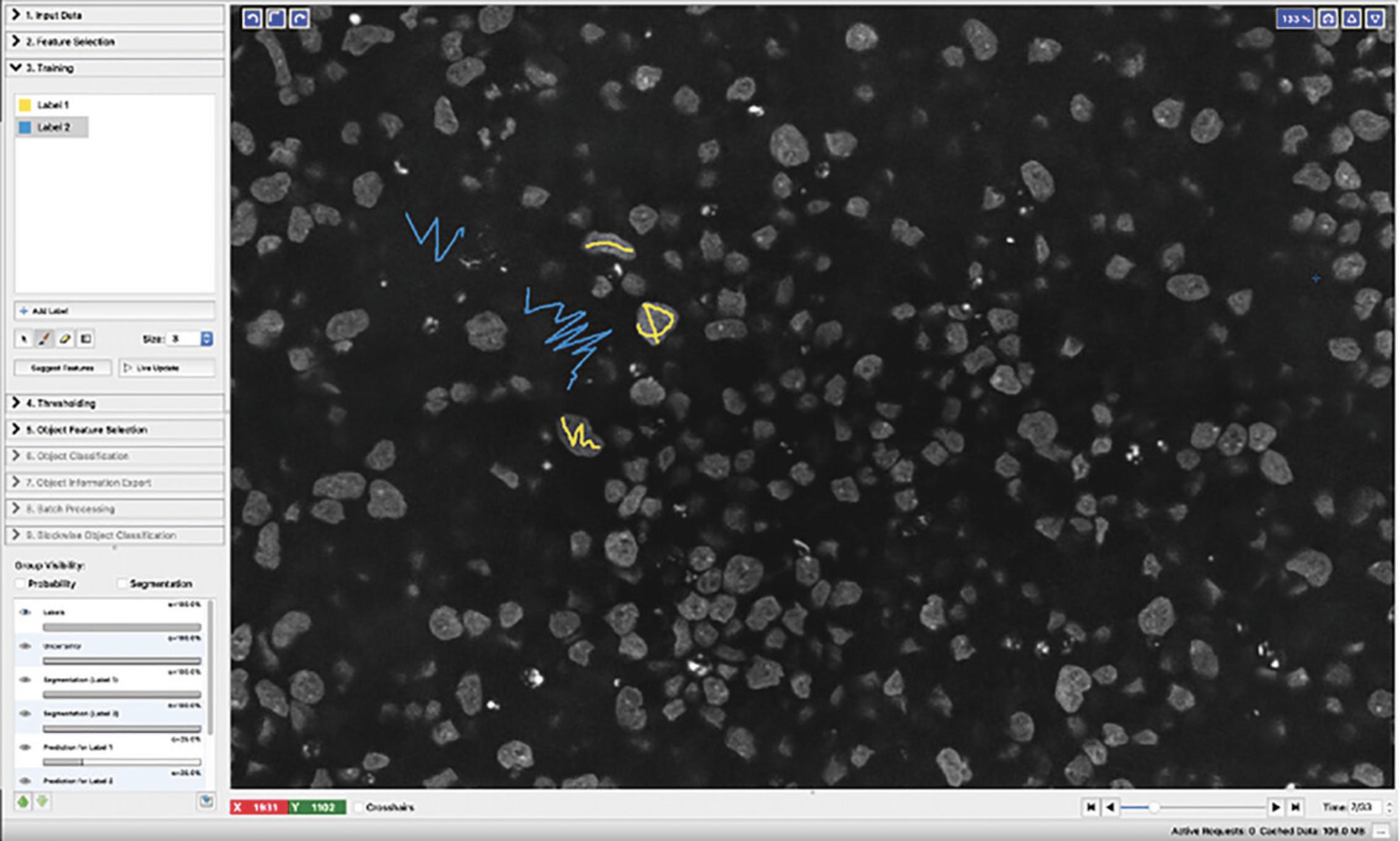
12.Click “Prediction Export” and change the Source setting under “Export Settings” to “Simple Segmentation.” Then click the “Choose Export Image Settings” button and change the “Output File Info Format” to “compressed hdf5” then click “OK.”
13.Click the Batch Processing tab on the left and then click the “Select Raw Data Files” button. Navigate to the round 1 image folder and highlight all TIF images from the first round of staining that correspond to the DAPI channel only. Click “Open.” Create the Ilastik masks by clicking “Process all files.”
14.Run “combineSegmentations.m” once the Ilastik masks and the Cellpose masks are completed and saved.
15.Run “SingleCellQuantification.m” to obtain single-cell measurements and write the data into CSV format. For your own experiment, change the name of the folders listed in the “dirs.” variable in line 10 to match the names of all the folders of the rounds in your data set.
Single cell analysis
16.Activate the Mplex_analysis environment if not already activated.
For Mac computers
-
Open the terminal.
-
Navigate to the “code” folder.
-
Enterconda activate Mplex_analysisto activate the environment, then enterjupyter labto launch Jupyterlab.
For Windows computers
-
d.Open the anaconda prompt.
-
e.Navigate to the “code” folder.
-
f.Create the Mplex_analysis environment before activating it.
-
conda env create -f Mplex_analysis_windows.yml
-
conda activate Mplex_analysis
-
g.Enter jupyter lab to launch JupyterLab.
17.Open ‘MultiplexedSCAnalysis.ipynb’ and begin executing the code blocks in the notebook and referenced in the following steps.
18.Import all required python packages.
- import scprep
- import numpy as np
- import pandas as pd
- import matplotlib.pyplot as plt
- import seaborn as sb
- import graphtools as gt
- from collections import Counter
- import seaborn as sns
- import tasklogger
- import sklearn
- import sklearn.cluster
- import sklearn.mixture
- import leidenalg as louvain
- import umap
- from sklearn.manifold import TSNE
- from sklearn.cluster import SpectralClustering
- import os
- from skimage import io as imio
- from skimage import exposure
- import scipy
- from scipy.stats import gaussian_kde
- from scipy.stats import pearsonr
19.Load the CSV file containing the processed image data using pandas.
20.Define lists that distinguish stain categories such as transcription factors that mark cell type, versus stains that measure cell signaling.
- marker_list = ['TFAP2C', 'TBXT', 'SOX17_1', 'ISL1', 'NANOG', 'SOX2', 'HAND1', 'OCT4', 'CDX2',
- 'MIXL1', 'PRDM1', 'FOXA2', 'OTX2', 'SNAI1', 'EOMES', 'TBX6']
- signal_list = ['cytopERK', 'pSmad1', 'BCAT', 'LEF1', 'ncSMAD2-3', 'ncYAP', 'cytopAKT']
- meta_list = ['nucArea', 'nucVolume', 'nucZ', 'Z', 'nucMajorAxis', 'nucMinorAxis', 'nucCircularity',
- 'nucSolidity', 'Colony', 'X', 'Y', 'RadialDist'] # 'nucOrientation' ,
- keep_list = ['DAPI_1'] + marker_list + signal_list + meta_list;
- data = raw_data[keep_list]
- data
21.Proceed running the code blocks Inspect Overlays (optional) and Quality Control.
22.Log transform the data.
23.Visualize the data using UMAP dimensionality reduction and cluster cell types using Louvain.
- # make UMAP based on marker genes alone
- # use min_dist to spread out points
- data_log_umap = umap.UMAP(min_dist=0.4).fit_transform(data_log[marker_list])
- data_log_umap = pd.DataFrame(data_log_umap, index=data_log.index)
- scprep.plot.scatter2d(data_log_umap, c=data_log['Colony'], label_prefix='UMAP',figsize=(5,5), ticks=False)
- plt.title('UMAP of marker genes')
- plt.show()
- # # louvain clustering with ONLY markers
- G = gt.Graph(data_log[marker_list])
- G_igraph = G.to_igraph()
- with tasklogger.log_task('Louvain'):
- partition = louvain.find_partition(G_igraph, louvain.RBConfigurationVertexPartition,
- weights='weight', resolution_parameter=0.2,seed=11)
- louvain_clusters = np.array(partition.membership)
- louvain_clusters
- data_log['Cluster'] = louvain_clusters
- scprep.plot.scatter2d(u_cdata, c=louvain_clusters, shuffle=False,
- title=curr_gene, ticks=None, label_prefix='UMAP',figsize=(7,7))
- plt.title('Louvain cluster over UMAP')
- plt.show()
Cell signaling analysis and cluster identification
24.Using the list of cell-signaling readouts, create heatmaps that show differential cell signaling or marker expression across the identified cell type clusters.
- # visualize
- cluster_means = pd.DataFrame(cluster_means, columns=data_log.columns[:-1],index=cluster_labels_reduced)
- cluster_means_zscore = pd.DataFrame(cluster_means_zscore, columns=data_log.columns[:-1],index=cluster_labels_reduced)
- row_linkage = scipy.cluster.hierarchy.linkage(cluster_means_zscore[signal_list], method='average');
- # show signaling heatmap
- g = sb.clustermap(cluster_means_zscore[signal_list], row_linkage=row_linkage,xticklabels=signal_list,vmax=2,vmin=-2,dendrogram_ratio=0.1,figsize=(10,5),cbar_pos=(0.01,0.01,0.03,0.2),cmap='RdBu_r')
- plt.setp(g.ax_heatmap.get_yticklabels(), rotation=0)
- plt.setp(g.ax_heatmap.get_xticklabels(), rotation=80)
- plt.show()
- # show marker heatmap
- g = sb.clustermap(cluster_means_zscore[marker_list], row_linkage=row_linkage,xticklabels=marker_list,vmax=2,vmin=-2,dendrogram_ratio=0.1,figsize=(10,5),cbar_pos=(0.01,0.01,0.03,0.2),cmap='RdBu_r')
- plt.setp(g.ax_heatmap.get_yticklabels(), rotation=0)
- plt.setp(g.ax_heatmap.get_xticklabels(), rotation=80)
- plt.show()
Spatial analysis
25.Run the ‘Spatial Analysis’ code blocks to visualize the distribution of clusters in space.
- # map the clusters back to space and overlay on DAPI image
- coli = 2; # colony index
- RGBoverlay = makeRGBoverlay(coli, ['DAPI']);
- plt.rcParams['figure.figsize']=(8,8)
- fig, ax = plt.subplots()
- ax.imshow(RGBoverlay, cmap='gray');
- ax.axis('off');
- for i in range(0,Nclusters):
- idx = (data_log['Colony']==coli) & (data_log['Cluster']==i);
- X = data_log.loc[idx]['X']
- Y = data_log.loc[idx]['Y']
- C = data_log.loc[idx]['Cluster']
- scatter = ax.scatter(X,Y,s=15, edgecolors='none');
-
- plt.legend(cluster_labels_reduced)
26.Run the Radial Density code block to plot the average cluster density versus radial edge-distance.
- # Cluster Radial Density
- plt.rcParams['figure.figsize']=(8,5)
- clusterdensities = {};
- r = np.linspace(0,350, num=350);
- clusterdensities_sum = 0*r;
- for i in range(0,Nclusters):
- idx = (data_log['Colony']==coli) & (data_log['Cluster']==i);
- R = data_log.loc[idx]['RadialDist']
- Z = gaussian_kde(R);
- Ncells = len(R);
- RZ = pd.concat([pd.Series(r),pd.Series(Z(r))],axis=1);
- RZ.rename(columns={0:'r',1:'Z'}, inplace=True);
- clusterdensity = NcellsRZ['Z']/(23.14159*r)
- clusterdensities[i] = clusterdensity;
- clusterdensities_sum = clusterdensities_sum + clusterdensity;
-
- for i in range(0,Nclusters):
- clusterfraction = clusterdensities[i]/clusterdensities_sum;
- plt.plot(RZ['r'], clusterfraction);
- plt.ylabel('fraction of cells');
- plt.xlabel('distance from center');
- plt.legend(cluster_labels_reduced,loc='lower left',bbox_to_anchor=(0.01, 0.1));
Support Protocol 1: GENERATING MICROPATTERNED MULTI-WELL SLIDES
This protocol describes how to generate micropatterned multi-well slides using photolithography. This relies on exposing a photosensitive substrate to a particular wavelength that changes the substrate's molecular structure. In this case, PLL-g-PEG, a hydrophobic photoresist to which neither cells nor proteins can adhere, is exposed to shortwave UV through a mask (Azioune et al., 2010). The specific areas where the PLL-g-PEG coated substrate is exposed to the UV light become hydrophilic and allow cell adherence. This method can be adapted to generate micropatterns of any desired geometry but requires the use of a custom designed photomask. We use 700 µm circular patterns with a pitch of 1100 µm to create 2D gastruloids, but CAD software can be utilized to customize the design of any photomask as needed.
Materials
- Ethanol
- HEPES (Sigma-Aldrich, cat. no. H4034-25G)
- MilliQ H2O
- PLL-g-PEG (PLL:20K-G:35-PEG:2K) (JenKem Technology)
PLL:20K-G:35-PEG:2K is a custom product with the formula: PEG-poly(L-lysine), poly(L-lysine), molecular weight of poly-lysine is 20,000 Da, molecular weight of mPEG is 2000 Da, graft ratio is 3.5+/−1.0, 100 mg.
-
Isopropanol
-
dH2O
-
Ultrasonic cleaner (Fosmon, cat. no. B07531F3GW)
-
Glass coverslips for sticky slides (ibidi, cat. no. 10812)
-
Bunsen burner
-
Container for coverslips
-
Biosafety cabinet
-
pH meter
-
Parafilm (Fisher Scientific, cat. no. 13-374-16)
-
10-cm plate
-
UV illuminator (Jelight Model 30 UVO cleaner)
-
Straight-edge stainless steel forceps
-
Plastic pipette tips
-
50-ml tube
-
Kimwipes (Fisher Scientific, cat. no. 06-666)
-
Fume hood
-
Photomask, chrome on quartz (Applied Image)
-
Paper towels
-
Sticky-slide 18 well (ibidi, cat. no. 81818-90)
-
Clamp and adapter for sticky slides (ibidi, cat. no. 80040 and 80045)
-
CAD software, e.g., AutoCAD 2022 (see Internet Resources)
-
Photomask design files, 700um_photomask.dxf and 700um_photomask.pdf (see Supporting Information)
Coat the glass coverslips with PLL-g-PEG
1.Add 200 ml 70% ethanol in the ultrasonic cleaner, add the coverslips to be coated and sonicate coverslips for 15 min.
2.Remove coverslips from the ultrasonic cleaner and flick away any ethanol. Pass them through a flame 3 to 4 times to sterilize.
3.Place coverslips in a clean container and bring inside the biosafety cabinet to ensure a sterile environment.
4.Make 10 mM HEPES buffer in MilliQ water and adjust pH to 7.45 to 7.50.
5.Make 1 mg/ml PLL-g-PEG solution in 10 mM HEPES buffer. Make 150 µl aliquots and freeze immediately.
6.Dilute an aliquot of 1 mg/ml PLL-g-PEG solution to a final concentration of 0.1 mg/ml in 10 mM HEPES solution.
7.Stretch new parafilm across a 10-cm plate (or other covered holder) and UV illuminate in the biosafety cabinet for 15 min.
8.Pipette 150 µl of 0.1 mg/ml PLL-g-PEG solution onto the parafilm.
9.Use straight edge forceps to carefully place a sterilized coverslip on top of the solution. Use plastic pipette tips to press down and remove any air bubbles to ensure an even coat.
10.Cover the holding plate and stretch parafilm across the outer edges to seal it. Leave in the hood for 4 hr.
11.Remove the coated coverslip from the holding plate and dunk twice in a 50-ml tube containing 45 to 50 ml of MilliQ water.
12.Flick away any excess water on a Kimwipe but be sure to keep the photoresist coated side of the coverslip face up and do not disturb the coating towards the center.
13.Aspirate any leftover solution from the parafilm in the holding plate and place the coated coverslips face up back in the holding plates.
14.Leave at room temperature until the next day.
Generate micropatterns by photolithography
15.Inside a fume hood, place the photomask chrome side up in a tray and add isopropanol until it is covered. Leave for 2 min.
16.Using straight-edge forceps, carefully remove the photomask from the tray and place on a paper towel. Place a large wet Kimwipe over top to remove excess isopropanol.
17.Submerge the photomask in dH2O for 2 min.
18.Remove from the dH2O and gently wipe down with a Kimwipe.
19.Place inside the UV illuminator, chrome side up.
20.Close UV illuminator and set for 10 min illumination.
21.Open the illuminator after the 10 min exposure and add 43 µl MilliQ H2O on top of the mask, chrome side up.
22.Slowly place the glass coverslip, coated side down on the mask being careful not to introduce air bubbles.
23.Flip the photomask so chrome side with coverslip is down and close the lid.
24.Illuminate for 12 min.
25.Remove photomask and submerge chrome side up in a tray with dH2O.
26.Wait 2 to 3 min until micropatterned coverslip floats to the top of the water. You may agitate the tray to speed this along.
27.Pick up the coverslip from the edge with forceps and flick away excess water.
28.Place the coverslip with the micropatterns face up on a Kimwipe.
29.Place coverslip back in the holding plate until assembly.
Assemble micropatterned multi-well slides
30.Inside the biosafety cabinet, open the individually packed sticky slide, remove its lid and place in the clamp adapter for 18-well sticky slides.
31.Remove the patterned glass coverslip with forceps and place on the flat part of the clamp adapter.
32.Remove the protective film from the sticky slide and fit adapter together then slide fitted adapter into the clamp.
33.Clamp tight for 2 min.
34.Remove the assembled micropatterned slide from the clamp, place its lid back on and place it inside the holding plate once more. Label the edge with serial number as required.
35.Store at 4°C.
Support Protocol 2: DIFFERENTIATION OF 2D GASTRULOIDS
This protocol describes how to seed and use micropatterned coverslips to generate micropatterned pluripotent stem cell colonies. The 2D micropatterned gastruloid model is a powerful tool for studying human gastrulation. This system allows for human-specific insights, with a high degree of reproducibility, while at the same time, it remains easily manipulatable (Deglincerti et al., 2016; Warmflash et al., 2014). We present this protocol for the 2D-gastruloid model, but the technique is widely useful for many developmental inquiries (Blin, 2021). Because the size and shape of micropatterns is customizable, micropatterning has been used in the study of organogenesis, including cardiac (Myers et al., 2013), liver (Kaylan et al., 2018), and pancreatic development (Tran et al., 2020).
Additional Materials (also see Basic Protocol 1 and Support Protocol 1)
-
Laminin-521 (Biolamina, cat. no. LN521)
-
DPBS, with Ca and Mg (Gibco, cat. no. 14-040-182)
-
Accutase (Fisher Scientific, cat. no. NC9464543)
-
mTeSR1 medium (Stemcell Technologies, cat. no. 85850)
-
ROCK inhibitor (RI) Y-27632 (MedChem Express, cat. no. HY-10583)
-
Human pluripotent stem cells (hPSCs), ESI-017 cell line (ESI BIO, cat. no. ES-700)
-
Recombinant human BMP-4 protein, CF (Fisher Scientific, cat. no. 314BP050CF)
-
10,000 U/ml penicillin-streptomycin (Fisher Scientific, cat. no. 15-140-148)
-
4% paraformaldehyde (PFA) fixative (see recipe)
-
Incubator, 37 ° C, 5% CO2
-
Benchtop centrifuge
-
Hemocytometer
-
Light microscope with 5× objective
Laminin coating micropatterns
1.Dilute laminin 1:20 (final 5 µg/ml) in room temperature DPBS.
2.Coat each well with 70 µl laminin solution and incubate 2 hr at 37°C.
3.Without removing the laminin solution, add 100 µl DPBS, then remove 100 µl from well(s).
4.Repeat this partial wash 3 more times.
5.Remove all solution from the well(s). Quickly pipette 100 µl DPBS in and out of the well(s).
6.Before the well(s) dry, replenish them with 100 µl DPBS for storage.
7.Store at 4°C.
Seeding cells onto micropatterns
8.Remove laminin coated plate from 4°C and place in cell incubator for 15 to 30 min.
9.Generate single cell suspension of pluripotent cells using accutase, spin the suspension 4 min at 1000 × g, room temperature, and resuspend the cells in mTeSR with 10 µM RI.
10.Count cells using a hemocytometer and seed 160,000 cells/0.34 cm2.
11.Move to the incubator for 35 min.
12.Remove medium, wash with PBS (without Ca and Mg).
13.Add mTeSR medium containing 10 µM RI. Do not let cells dry out.
14.Check under the microscope to confirm intact patterns without holes.
15.After 1 hr, change medium for 200 µl/well medium containing 50 ng/ml BMP-4 + 100 U/ml penicillin-streptomycin without RI.
16.Incubate cells at 37 ° C and 5% CO2 for 42 hr or 48 hr after addition of BMP-4.
Fixation
17.Wash 2 times with 150 µl PBS (without Ca and Mg).
18.Fix with 70 μl of 4% PFA/well for 20 min.
19.Wash 2 times with 150 µl PBS (without Ca and Mg).
20.Store in PBS with 0.01% sodium azide at 4°C until ready to stain.
21.Continue to step 2 in Basic Protocol 1 for staining instructions.
REAGENTS AND SOLUTIONS
Antibody solution, primary
Determine volume by number of wells to stain in the experiment. Dilute primary antibodies (customizable; see Table 1) in donkey block (+ Triton X-100 in round 1 of staining and – Triton X-100 after round 1; see recipe). Prepare fresh.
Antibody solution, secondary
Determine volume by number of wells to stain in the experiment. Dilute secondary antibodies (customizable; see Table 2) in donkey block (+ Triton X-100 in round 1 of staining and – Triton X-100 after round 1; see recipe). Add 1 μg/ml DAPI (Thermo Fisher Scientific, cat. no. D1306). Prepare fresh.
Antigen | Manufacturer | Cat. no. | Dilution |
---|---|---|---|
Donkey anti-mouse Alexa Fluor 488 | Thermo Fisher Scientific | A21202 | 1/500 |
Donkey anti-rabbit Alexa Fluor 555 | Thermo Fisher Scientific | A31572 | 1/500 |
Donkey anti-goat Alexa Fluor 647 | Thermo Fisher Scientific | A21447 | 1/500 |
Donkey anti-rabbit Alexa Fluor 647 | Thermo Fisher Scientific | A31573 | 1/500 |
Donkey anti-goat Alexa Fluor 555 | Thermo Fisher Scientific | A21432 | 1/500 |
Donkey anti-rat Alexa Fluor 555 | Abcam | ab150154 | 1/500 |
Donkey anti-rabbit Alexa Fluor 488 | Thermo Fisher Scientific | A21206 | 1/500 |
Staining round | Secondary antibody dye 488 | Laser (%)/exposure (ms) | Secondary antibody dye 555 | Laser (%)/exposure (ms) | Secondary antibody dye 647 | Laser (%)/exposure (ms) |
---|---|---|---|---|---|---|
1 |
SMAD2-3 (mouse) |
8 150 |
pAKT (rabbit) |
10 150 |
SOX17 (goat) |
5 50 |
2 |
GATA3 (mouse) |
8 120 |
OTX2 (goat) |
5 60 |
LEF1 (rabbit) |
7 130 |
3 |
ISL (mouse) |
5 40 |
TBXT (goat) |
5 60 |
pSMAD1/5/9 (rabbit) |
6 100 |
4 |
EOMES (mouse) |
7 50 |
YAP (rabbit) |
8 50 |
FOXA2 (goat) |
8 200 |
5 |
TFAP2C (mouse) |
9 80 |
NANOG (goat) |
8 60 |
pERK (rabbit) |
10 150 |
6 |
BCAT (mouse) |
6 80 |
TBX6 (goat) |
7 50 |
SMAD2 (rabbit) |
10 150 |
7 |
SOX2 (rabbit) |
6 50 |
PRDM1 (rat) |
10 200 |
SOX17 (goat) |
2 30 |
8 |
OCT3/4 (mouse) |
10 150 |
SMAD1 (rabbit) |
10 150 |
HAND1 (goat) |
5 30 |
9 |
CDX2 (mouse) |
10 150 |
MIXL1 (rabbit) |
5 30 |
SNAI1 (goat) |
5 30 |
Donkey block
For 1 ml:
- 30 µl donkey serum (Sigma-Aldrich, cat. no. S30-M)
- 10 µl penicillin-streptomycin (Fisher Scientific, cat. no. 15-140-148)
- 959 μl PBS, without Ca and Mg (Corning, cat. no. 21-040-CV)
- 1 µl Triton X-100 (Millipore Sigma, cat. no. TX1568-1)
- Prepare fresh
Only use Triton X-100 in the first round of staining. Do not include Triton X-100 in any solutions after round 1.
Elution buffer (EB), pH 2.5
For 20 ml:
- 12 ml ddH2O
- 0.750 g L-glycine (Sigma-Aldrich, cat. no. G7126-100G)
- 3.604 g urea (Sigma-Aldrich, cat. no. U5378-100G)
- 5.772 g guanidine hydrochloride (Sigma-Aldrich, cat. no. 63272-100G)
- 0.400 g Tris(2-carboxyethyl)phosphine hydrochloride (TCEP) (Sigma-Aldrich, cat. no. C4706-2G)
- Adjust pH to 2.5 with HCl or NaOH
- Store up to 3 weeks at 4°C
Prepare under chemical fume hood.
Imaging buffer (IB), pH 7.4
For 25 ml:
- 6 ml ddH2O
- 2.76 g N-acetyl-l-cysteine (NAC) (Sigma-Aldrich, cat. no. A7250-50G)
- 17 ml 1 N NaOH (Fisher Chemical, cat. no. SS266-1)
- Adjust pH to 7.4 with HCl or NaOH
- Store protected from light up to 2 weeks at room temperature
Prepare under chemical fume hood.
PBST
For 1 ml:
- 999 μl PBS, without Ca and Mg (Corning, cat. no. 21-040-CV)
- 1 μl Tween 20 (Fisher Scientific, cat. no. BP337-500)
- Prepare fresh
PFA fixative, 4%
For 1.5 ml:
- 375 µl 16% PFA (Fisher Scientific, cat. no. 50-980-487)
- 150 µl 10X DPBS without Ca and Mg (Corning, cat. no. 20-032-CV)
- 975 µl H2O, molecular biology grade (IBI Scientific, cat. no. IB42111)
Prepare under chemical fume hood.
COMMENTARY
Background Information
Spatial transcriptomics methods promise transcriptome-wide characterization of RNA expression with single cell resolution and spatial context (Method of the Year 2020: Spatially Resolved Transcriptomics, 2021; Wang et al., 2023). However, many questions can only be answered with information obtained from direct imaging of proteins, particularly when post-translational modifications and/or protein localization at the cellular and tissue level are of interest. How cellular signaling activity relates to differentiation of cells within the spatial context of tissues is one such question. In differentiating tissues, the activity of many cell-signaling pathways can be inferred from changes in localization (e.g., cytoplasmic to nuclear translocation) or post-translational modifications (e.g., phosphorylation). Although there are transcriptional correlates of signaling activity that are sometimes used as a proxy for signaling activity in transcriptomic data (e.g., axin2 for Wnt signaling), this assumption of correlation between transcript level and signaling activity is not valid across all genes and experimental contexts (Barolo, 2006; Heemskerk et al., 2019).
Highly multiplexed immunofluorescence staining combined with high resolution imaging enables obtaining single cell expression profiles for large numbers of proteins while preserving spatial context. However, traditional IF has been limited to a few antibody targets per sample. Overcoming this issue either involves repeated staining and imaging or circumventing both spectral overlap and antibody cross-reactivity (Gendusa et al., 2014). Cross-reactivity can be bypassed using direct immunofluorescence with antibodies pre-conjugated to fluorophores or using an indirect approach that does not rely on secondary antibodies, such as oligonucleotide-conjugated antibodies. For example, CODEX involves a single step of staining with oligonucleotide-conjugated antibodies that are revealed in cycles of imaging and fluorophore removal (Black et al., 2021; Goltsev et al., 2018). However, this requires specialized reagents and equipment. More generally, direct immunofluorescence does not allow signal amplification resulting in lower signal to noise and additionally requires the creation of conjugated antibody panels (Hickey et al., 2022). Even with these techniques, spectral overlap remains a challenge, limiting the number of proteins that can be imaged at a time. Spectral overlap can be partially overcome using hyperspectral imaging, but this approach still limits the number of markers to ∼20, is technically challenging, and requires antibodies directly conjugated to a large number of distinct dyes (Gerner et al., 2012; Stack et al., 2014). Thus, these approaches are highly technically demanding, expensive, and remain limited in signal to noise.
Alternatively, both problems can be avoided with standard equipment and simple reagents by taking a fully iterative approach. Techniques that both image and stain in cycles include: 4i, eMAP, t-CyCIF, and IBEX (Gut et al., 2018; Park et al., 2021; Lin et al., 2018; Radtke et al., 2022). These methods are distinguished by several factors, such as signal clearing between cycles (either bleaching or antibody removal), direct or indirect immunofluorescence, and chemical reagents. Fully iterative methods typically have stronger analytical requirements, including image registration and image segmentation. We selected the 4i technique from which to build the current method and pipeline because it is an indirect IF technique that only requires a few relatively inexpensive chemicals beyond all the other materials that a cell biology lab would typically already possess to perform IF. Moreover, we found it to be compatible with the majority of our antibodies and the signal removal by elution was highly efficient. The original protocol by Gut et al. (2018) was adapted here for use on 2D gastruloids, and we further developed a custom image analysis pipeline in Basic Protocol 2 to enable automated image segmentation, registration, and co-localization analyses for tissues with densely packed, multilayered nuclei.
Our approach enables relating cell fate to highly combinatorial signaling activity in 2D gastruloids. More generally, by simultaneously revealing spatial heterogeneity in cell fate and signaling, our pipeline enables insight into the origin of heterogeneous differentiation under homogeneous media conditions, which is a major obstacle for applications of stem cell technology. Each approach for mapping tissue biology involves a tradeoff between depth of multiplexing, spatial information, temporal information, and modalities (i.e., protein, RNA). One major limitation of the technique presented here is that cells are fixed, so temporal information is missing, although dynamics might be inferred from snapshots given certain assumptions (Ding et al., 2022; Saelens et al., 2019; Serra et al., 2019). Another limitation is the limited depth of gene coverage compared to transcriptomics methods (around 30 in multiplexed IF compared to hundreds or even thousands in scRNA-seq). However, this method is uniquely suited for visualizing spatial localization of many proteins, as is required not only for relating signaling to fate, but also for studies of tissue mechanics and cell migration.
Critical Parameters
hPSC cell culture and differentiation
While micropatterning greatly increases robustness of results in the 2D human gastruloid model, it should be noted that quality of cell culture and cell culture technique is paramount to obtaining robust results. Factors such as cell line variation, cell density just before passaging, micropatterned cell density, and incubation parameters can cause variability in results.
Quality control of image alignment and cell alignment
The quality of the data must be inspected before proceeding to full analysis. It is critical that images from every round of staining are in focus, completely captured in z, properly stitched, and aligned. A first impression of image quality and stitching can be obtained during acquisition and is described in Basic Protocol 1 step 24. Alignment can be checked by inspection of overlays from different rounds as described in Basic Protocol 2 step 3. For detecting more subtle errors resulting from the image data, we implemented a self-correlation plot as the quality control measure to ascertain that there is a strong correlation between the selected stain in different rounds. In this example, we measure SOX17 expression once in the first round of staining, and again in the 7th round. If the self-correlation of cells between the first and last images is strong (typically >0.95) it is reasonable to conclude that the whole pipeline functions properly. This is demonstrated in Figure 7, which compares colonies that were or were not pre-treated with EB; tissue deformation due to EB leads to misalignment between cells in different rounds without EB pre-treatment even if images are optimally aligned and stitched.
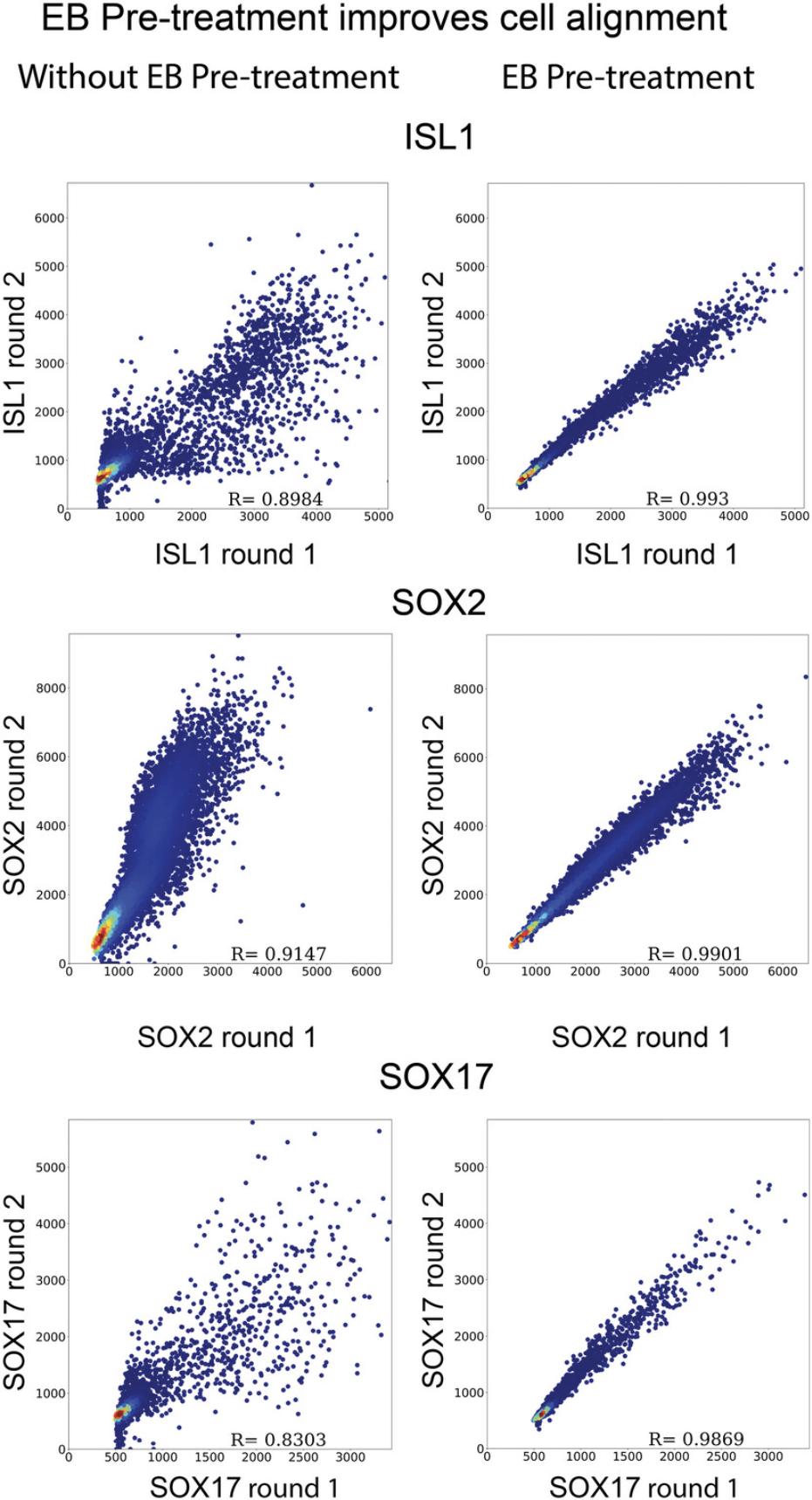
Cell signaling metrics
When analyzing cell signaling, it is important to use the correct readout of cell signaling. For example, SMAD2 translocates to the nucleus during active Nodal signaling. The relevant readout for this type of signaling is the nuclear to cytoplasmic ratio (stored in the variable “ncSMAD2-3”). While for ERK activity, we use the cytoplasmic measurement, “cytopERK,” since pERK is mostly cytoplasmic.
Troubleshooting
Troubleshooting in Table 4 shows potential solutions to commonly encountered problems.
Problem | Possible cause | Solution |
---|---|---|
Poor elution | Antibody concentration is too high | If stain is very bright, it may be possible to dilute the antibody more so that the stain is still good quality but elutes better |
Failure to use IB/exposure to light without IB | Make certain that the stained sample is not exposed to light unless it is in IB | |
Imaging settings are too high | Some stains require higher laser power and longer exposure, which increases the risk of incomplete elution; reduce power or exposure if possible | |
Cells detaching | Pipette technique is too aggressive | Gently pipette dropwise when adding medium to fixed cells and pipette up slowly when removing medium |
Nonsensical clustering results | Outlier intensity values due to junk staining | At the time of imaging, wash the well a few more times to dislodge and remove the junk |
Clustering resolution is improperly set | Adjust the resolution parameter during Louvain clustering | |
Poor quality micropatterned colonies | UV illumination not right | Adjust water droplet size between mask and slide |
Check that UV exposure time is correct, and that illuminator stage is not tilted | ||
Check for UV lamp expiration and replace bulb if needed | ||
Inadequate laminin coating | Prevent the well from drying out during coating or adjust laminin incubation time | |
Too much or too little washing of cells after seeding | Optimize pipetting technique to remove non-specifically bound cells without damaging micropatterned colonies | |
Quantification results in cells with intensity values of NaN | During imaging, some cells are incompletely imaged in the z plane | During imaging, set the z limits so the entirety of all cells is imaged |
Understanding Results
Proper execution of the protocols mentioned herein will result in several key plots (as shown in Fig. 1): cell fate UMAP showing cell type clusters, heat maps of gene expression and signaling by cluster, and spatial analysis that identifies cell type organization in space. As an example, we share results from the micropatterned 2D human gastruloid model at 48 hr of differentiation. Cell fate clustering revealed 7 cell types: pluripotent, ectoderm-like cells, incipient mesoderm-like cells, amnion-like cells, primordial germ cell-like cells, primitive streak-like cells, and mitotic cells. These cell types are consistent with single cell RNA-sequencing data for a similar differentiation experiment in Jo et al. (2022). In general, we suggest using reference scRNA-seq data or searching existing literature to choose marker genes to stain for and then comparing clusters from multiplex IF data with those from scRNA-seq data or cell types described in the literature to make sure the results are sensible. Using image-based analysis, the spatial relationship of cell fates and the relationship between fate and signaling can then also be investigated. For example, here we mapped clusters back to their spatial position and plotted the prevalence of each cell types as a function of distance from the colony edge. Similarly, signaling profiles for each cluster were extracted, showing for example that pSMAD1 and nuclear YAP are highest in amnion-like cells. The results are consistent with what had been previously pieced together from many different stains across papers, but now come from a single experiment that can be further mined for correlations between stains at the single cell level.
Time Considerations
The staining step takes ∼5 hr but is mostly passive as most of the time is spent incubating stains. With our setup, it takes ∼10 min to image a 700 µm diameter colony in a 6 × 4 grid at 40× with approximately 30 z-steps and 4 channels. We typically image 6 colonies per well which takes ∼1 hr per well. Hence, it is advisable to complete staining and imaging for one round in 1 day and then to store the sample in PBS with sodium azide overnight and continue the experiment the next morning. Each planned round of staining should be expected to take a day; thus a 10-round plan will take 10 days. The amount of time needed for the computational analysis is dependent on the specific experiment and the specifications of the computer. Rough estimates for the example experiment described in this article (i.e., 6 colonies, 9 rounds), using an iMac with a 4 GHz Quad-Core Intel Core i7 processor, are as follows: alignment ∼3 hr, Ilastik segmentation 30 min of user input, ∼1.5 hr batch processing, Cellpose segmentation 3 hr, combining segmentations 20 min, single cell quantification 1 hr, single cell analysis ∼5 to 10 min. Most of the computational steps do not require user input beyond setting them up and executing the scripts, therefore the time estimates reflect processing time where the user is free to perform other tasks.
Acknowledgments
This work was funded by the National Institute of General Medical Sciences (NIGMS R35GM138346), National Science Foundation RECODE (2033654), and National Institutes of Health Cellular Biotechnology Training Program (CBTP) T32GM145304.
Author Contributions
Emily Freeburne : Conceptualization; data curation; formal analysis; investigation; methodology; software; validation; visualization; writing original draft; Writing review and editing. Seth Teague : Conceptualization; data curation; formal analysis; investigation; methodology; software; validation; visualization; writing review and editing. Hina Khan : Data curation; investigation; methodology; writing review and editing. Bolin Li : Conceptualization; data curation; investigation; methodology; software. Siyuan Ding : Formal analysis; investigation; methodology; software; visualization. Bohan Chen : Investigation. Adam Helms : writing review and editing. Idse Heemskerk : Conceptualization; data curation; formal analysis; funding acquisition; methodology; project administration; resources; software; supervision; validation; visualization; writing original draft; writing review and editing.
Conflict of Interest
The authors declare no conflict of interest.
Open Research
Data Availability Statement
Raw data for 2 rounds of staining of two colonies are included to test the image processing pipelines. Due to limitations in file size by the journal, these can be found in the data subfolder at: https://www.dropbox.com/sh/edz56lj4teyb1pq/AACPhzhPmPCoozVprSSsDttWa?dl=0
A processed intensity value matrix for a full experiment and maximal intensity projections for overlays of 3 rounds of staining are included as supplementary files to test single cell analysis methods. Scripts for image analysis included as supplementary files and at github.com/idse/6i.
Supporting Information
Filename | Description |
---|---|
700umphotomask.dxf79.3 MB | |
700umphotomask.pdf19.6 MB | Photomask design files. |
230702CPJOBS.bin42.6 KB | |
code.zip5 GB |
Please note: The publisher is not responsible for the content or functionality of any supporting information supplied by the authors. Any queries (other than missing content) should be directed to the corresponding author for the article.
Literature Cited
- Azioune, A., Carpi, N., Tseng, Q., Théry, M., & Piel, M. (2010). Protein micropatterns: A direct printing protocol using deep UVs. Methods in Cell Biology , 97, 133–146. https://doi.org/10.1016/S0091-679X(10)97008-8
- Barolo, S. (2006). Transgenic Wnt/TCF pathway reporters: All you need is Lef? Oncogene , 25(57), 7505–7511. https://doi.org/10.1038/sj.onc.1210057
- Berg, S., Kutra, D., Kroeger, T., Straehle, C. N., Kausler, B. X., Haubold, C., Schiegg, M., Ales, J., Beier, T., Rudy, M., Eren, K., Cervantes, J. I., Xu, B., Beuttenmueller, F., Wolny, A., Zhang, C., Koethe, U., Hamprecht, F. A., & Kreshuk, A. (2019). ilastik: Interactive machine learning for (bio)image analysis. Nature Methods , 16(12), 1226–1232. https://doi.org/10.1038/s41592-019-0582-9
- Black, S., Phillips, D., Hickey, J. W., Kennedy-Darling, J., Venkataraaman, V. G., Samusik, N., Goltsev, Y., Schürch, C. M., & Nolan, G. P. (2021). CODEX multiplexed tissue imaging with DNA-conjugated antibodies. Nature Protocols , 16(8), 3802–3835. https://doi.org/10.1038/s41596-021-00556-8
- Blin, G. (2021). Quantitative developmental biology in vitro using micropatterning. Development , 148(15), dev186387. https://doi.org/10.1242/dev.186387
- Chhabra, S., Liu, L., Goh, R., Kong, X., & Warmflash, A. (2019). Dissecting the dynamics of signaling events in the BMP, WNT, and NODAL cascade during self-organized fate patterning in human gastruloids. PLOS Biology , 17(10), e3000498. https://doi.org/10.1371/journal.pbio.3000498
- Deglincerti, A., Etoc, F., Guerra, M. C., Martyn, I., Metzger, J., Ruzo, A., Simunovic, M., Yoney, A., Brivanlou, A. H., Siggia, E., & Warmflash, A. (2016). Self-organization of human embryonic stem cells on micropatterns. Nature Protocols , 11(11), 2223–2232. https://doi.org/10.1038/nprot.2016.131
- Ding, J., Sharon, N., & Bar-Joseph, Z. (2022). Temporal modelling using single-cell transcriptomics. Nature Reviews Genetics , 23(6), 355–368. https://doi.org/10.1038/s41576-021-00444-7
- Gendusa, R., Scalia, C. R., Buscone, S., & Cattoretti, G. (2014). Elution of high-affinity (>10 -9 K D) antibodies from tissue sections: Clues to the molecular mechanism and use in sequential immunostaining. Journal of Histochemistry & Cytochemistry, 62(7), 519–531. https://doi.org/10.1369/0022155414536732
- Gerner, M. Y., Kastenmuller, W., Ifrim, I., Kabat, J., & Germain, R. N. (2012). Histo-cytometry: A method for highly multiplex quantitative tissue imaging analysis applied to dendritic cell subset microanatomy in lymph nodes. Immunity , 37(2), 364–376. https://doi.org/10.1016/j.immuni.2012.07.011
- Goltsev, Y., Samusik, N., Kennedy-Darling, J., Bhate, S., Hale, M., Vazquez, G., Black, S., & Nolan, G. P. (2018). Deep profiling of mouse splenic architecture with CODEX multiplexed imaging. Cell , 174(4), 968–981.e15. https://doi.org/10.1016/j.cell.2018.07.010
- Gut, G., Herrmann, M. D., & Pelkmans, L. (2018). Multiplexed protein maps link subcellular organization to cellular states. Science , 361(6401), eaar7042. https://doi.org/10.1126/science.aar7042
- Heemskerk, I., Burt, K., Miller, M., Chhabra, S., Guerra, M. C., Liu, L., & Warmflash, A. (2019). Rapid changes in morphogen concentration control self-organized patterning in human embryonic stem cells. ELife , 8, e40526. https://doi.org/10.7554/eLife.40526
- Heemskerk, I. (2020). Full of potential: Pluripotent stem cells for the systems biology of embryonic patterning. Systems Biology of Pattern Formation , 460(1), 86–98. https://doi.org/10.1016/j.ydbio.2019.05.004
- Hickey, J. W., Neumann, E. K., Radtke, A. J., Camarillo, J. M., Beuschel, R. T., Albanese, A., McDonough, E., Hatler, J., Wiblin, A. E., Fisher, J., Croteau, J., Small, E. C., Sood, A., Caprioli, R. M., Angelo, R. M., Nolan, G. P., Chung, K., Hewitt, S. M., Germain, R. N., … Saka, S. K. (2022). Spatial mapping of protein composition and tissue organization: A primer for multiplexed antibody-based imaging. Nature Methods , 19(3), 284–295. https://doi.org/10.1038/s41592-021-01316-y
- Jo, K., Teague, S., Chen, B., Khan, H. A., Freeburne, E., Li, H., Li, B., Ran, R., Spence, J. R., & Heemskerk, I. (2022). Efficient differentiation of human primordial germ cells through geometric control reveals a key role for Nodal signaling. ELife , 11, e72811. https://doi.org/10.7554/eLife.72811
- Kagiwada, S., Aramaki, S., Wu, G., Shin, B., Kutejova, E., Obridge, D., Adachi, K., Wrana, J. L., Hübner, K., & Schöler, H. R. (2021). YAP establishes epiblast responsiveness to inductive signals for germ cell fate. Development , 148(20), dev199732. https://doi.org/10.1242/dev.199732
- Kaylan, K. B., Berg, I. C., Biehl, M. J., Brougham-Cook, A., Jain, I., Jamil, S. M., Sargeant, L. H., Cornell, N. J., Raetzman, L. T., & Underhill, G. H. (2018). Spatial patterning of liver progenitor cell differentiation mediated by cellular contractility and Notch signaling. ELife , 7, e38536. https://doi.org/10.7554/eLife.38536
- Lin, J. R., Izar, B., Wang, S., Yapp, C., Mei, S., Shah, P. M., Santagata, S., & Sorger, P. K. (2018). Highly multiplexed immunofluorescence imaging of human tissues and tumors using t-CyCIF and conventional optical microscopes. eLife , 7, e31657. https://doi.org/10.7554/eLife.31657
- Method of the Year 2020: spatially resolved transcriptomics. (2021). Nature Methods , 18(1), 1. https://doi.org/10.1038/s41592-020-01042-x
- Myers, F. B., Silver, J. S., Zhuge, Y., Beygui, R. E., Zarins, C. K., Lee, L. P., & Abilez, O. J. (2013). Robust pluripotent stem cell expansion and cardiomyocyte differentiation via geometric patterning. Integrative Biology , 5(12), 1495–1506. https://doi.org/10.1039/c2ib20191g
- Pachitariu, M., & Stringer, C. (2022). Cellpose 2.0: How to train your own model. Nature Methods , 19(12), 1634–1641. https://doi.org/10.1038/s41592-022-01663-4
- Park, J., Khan, S., Yun, D. H., Ku, T., Villa, K. L., Lee, J. E., Zhang, Q., Park, J., Feng, G., Nedivi, E., & Chung, K. (2021). Epitope-preserving magnified analysis of proteome (eMAP). Science Advances , 7(46), eabf6589. https://doi.org/10.1126/sciadv.abf6589
- Radtke, A. J., Chu, C. J., Yaniv, Z., Yao, L., Marr, J., Beuschel, R. T., Ichise, H., Gola, A., Kabat, J., Lowekamp, B., Speranza, E., Croteau, J., Thakur, N., Jonigk, D., Davis, J. L., Hernandez, J. M., & Germain, R. N. (2022). IBEX: An iterative immunolabeling and chemical bleaching method for high-content imaging of diverse tissues. Nature Protocols , 17(2), 378–401. https://doi.org/10.1038/s41596-021-00644-9
- Saelens, W., Cannoodt, R., Todorov, H., & Saeys, Y. (2019). A comparison of single-cell trajectory inference methods. Nature Biotechnology , 37(5), 547–554. https://doi.org/10.1038/s41587-019-0071-9
- Serra, D., Mayr, U., Boni, A., Lukonin, I., Rempfler, M., Challet Meylan, L., Stadler, M. B., Strnad, P., Papasaikas, P., Vischi, D., Waldt, A., Roma, G., & Liberali, P. (2019). Self-organization and symmetry breaking in intestinal organoid development. Nature , 569(7754), 66–72. https://doi.org/10.1038/s41586-019-1146-y
- Stack, E. C., Wang, C., Roman, K. A., & Hoyt, C. C. (2014). Multiplexed immunohistochemistry, imaging, and quantitation: A review, with an assessment of Tyramide signal amplification, multispectral imaging and multiplex analysis. Advancing the Boundaries of Molecular Cellular Pathology , 70(1), 46–58. https://doi.org/10.1016/j.ymeth.2014.08.016
- Tran, R., Moraes, C., & Hoesli, C. A. (2020). Controlled clustering enhances PDX1 and NKX6.1 expression in pancreatic endoderm cells derived from pluripotent stem cells. Scientific Reports , 10(1), 1190. https://doi.org/10.1038/s41598-020-57787-0
- Wang, Y., Liu, B., Zhao, G., Lee, Y., Buzdin, A., Mu, X., Zhao, J., Chen, H., & Li, X. (2023). Spatial transcriptomics: Technologies, applications and experimental considerations. Genomics , 115, 110671. https://doi.org/10.1016/j.ygeno.2023.110671
- Warmflash, A., Sorre, B., Etoc, F., Siggia, E. D., & Brivanlou, A. H. (2014). A method to recapitulate early embryonic spatial patterning in human embryonic stem cells. Nature Methods , 11(8), 847–854. https://doi.org/10.1038/nmeth.3016
Internet Resources
Download source for Ilastik.
Anaconda.
Source for AutoCAD 2022.
Citing Literature
Number of times cited according to CrossRef: 1
- Seth Teague, Gillian Primavera, Bohan Chen, Zong-Yuan Liu, LiAng Yao, Emily Freeburne, Hina Khan, Kyoung Jo, Craig Johnson, Idse Heemskerk, Time-integrated BMP signaling determines fate in a stem cell model for early human development, Nature Communications, 10.1038/s41467-024-45719-9, 15 , 1, (2024).