Single-Nucleus RNA-Sequencing in Brain Tissue
Johannes Bohacek, Johannes Bohacek, Rebecca Waag, Rebecca Waag
Abstract
Neuroscience research greatly benefits from single-cell sequencing technologies, which can reveal transcriptional alterations on a cellular level. However, preparing single-cell suspensions is technically challenging, requires experience, and has several limitations that can influence the transcriptional readout. Performing sequencing of single nuclei instead of single cells alleviates several of the challenges of sample preparation and highlights acute nuclear transcription. Here, we provide a protocol to prepare a nuclei suspension for single-nucleus RNA-sequencing for cell type-specific transcriptional profiling of brain tissue using the 10x Genomics single-cell gene expression assay. Furthermore, we highlight important aspects to consider during experimental design and data analysis. © 2023 The Authors. Current Protocols published by Wiley Periodicals LLC.
Basic Protocol 1 : Preparation of single-nucleus suspension
Basic Protocol 2 : Preparation and sequencing of single-nucleus libraries for RNA-seq
INTRODUCTION
Transcriptomic analyses are widely used to quantify gene expression as a proxy for the activity of genes and pathways in response to experimental interventions. However, bulk transcriptional analyses fail to fully resolve the complexity underlying the transcriptional response as they average all transcriptional information present in the tissue. This constitutes a major challenge in the field of neuroscience given the huge cellular diversity within the central nervous system. The advent of single-cell technologies, allowing a transcriptional readout at the single cell level (Tang et al., 2009), was thus quickly adopted in the field and has increased in popularity ever since (Svensson et al., 2018). The technique not only allows tracing transcriptional signals back to specific cell types but can also reveal treatment effects that are hidden in a bulk readout. Treatment effects could be hidden in bulk, if the effect occurs in a very scarce cell type (e.g., endothelial cells make up a small part of the entire transcriptome), or if individual genes are regulated in opposite directions in different cell types, effectively neutralizing each other in the bulk signal.
The quality of any single-cell data depends on the ability to dissociate the tissue into viable single units, such as cells. Therefore, highly interconnected tissues like the brain constitute a major challenge for successful dissociation. Obtaining high quality viable cells from the brain has proven difficult, and current protocols are limited by major drawbacks (Bakken et al., 2018). First, fresh tissue must be used to obtain viable cells, which can place considerable constraints on the experimental design (e.g., challenging parallel processing of several samples, thus rendering high throughput experiments impossible). In addition, commonly used tissue dissociation protocols rely on enzymatic tissue dissociation at high temperatures, which can trigger artificial upregulation of certain transcripts, thus confounding the gene expression results (Lacar et al., 2016; van den Brink et al., 2017). Several adjustments to the dissociation process, such as the use of cold-active enzymes, dissociation at lower temperatures, or the use of transcription inhibitors during dissociation have been shown to alleviate these artifacts (Adam et al., 2017; Marsh et al., 2022; Wu et al., 2017).
The use of nuclei instead of whole cells constitutes a valuable alternative that circumvents several of the drawbacks of single-cell protocols (Grindberg et al., 2013; Lake et al., 2017). First, the ability to extract nuclei from frozen tissue allows flash-freezing of the tissue upon collection, thus affording greater flexibility during experimental design and high-throughput tissue processing. Additionally, as multiple samples can be processed together, the risk of batch effects can be minimized. Further, no enzymatic dissociation step is required, thus all steps of the protocol can be performed on ice, which is critical to obtain high-quality transcriptional data with minimal dissociation-induced artifacts (Denisenko et al., 2020; Lacar et al., 2016). In peripheral tissues, it has also been shown that the use of nuclei preserved sensitive cells better, thus providing a better capture of cell type diversity (Wu et al., 2019). It is important to consider, however, that data obtained from single-nucleus RNA-sequencing (snRNA-seq) differs from single-cell RNA-seq (scRNA-seq). While scRNA-seq can capture all mRNAs within a cell, data from snRNA-seq misses non-nuclear RNAs. Therefore, snRNA-seq data is dominated by nascent transcripts, including intronic reads (Bakken et al., 2018). This offers an additional advantage as it gives a clearer picture of ongoing transcription without being blurred by mature transcripts. Clustering into cellular subpopulations is preserved as a majority of marker genes is maintained (Bakken et al., 2018; Kalish et al., 2020; Lake et al., 2017). From a scientific point of view, the use of nuclei can be combined with epigenetic techniques, e.g., assay for transposase-accessible chromatin using sequencing (ATAC-seq) (Buenrostro et al., 2013), thus providing a rich, multi-omic readout for each individual nucleus (Dimitriu et al., 2022). Finally, in the context of translational neuroscience, results from single-nucleus studies can be readily compared to human clinical samples, which are typically stored frozen (Cuevas-Diaz Duran et al., 2022; Maitra et al., 2021).
Here, we provide protocols for the preparation of a single-nucleus suspension and of libraries for snRNA-seq (Fig. 1). We address common problems and offer tips along each step to obtain high quality data. While the protocol for the preparation of a nucleus suspension was designed to be used for snRNA-seq using the 10x Genomics platform, it is also applicable to a diverse set of downstream techniques that require single nuclei as input.
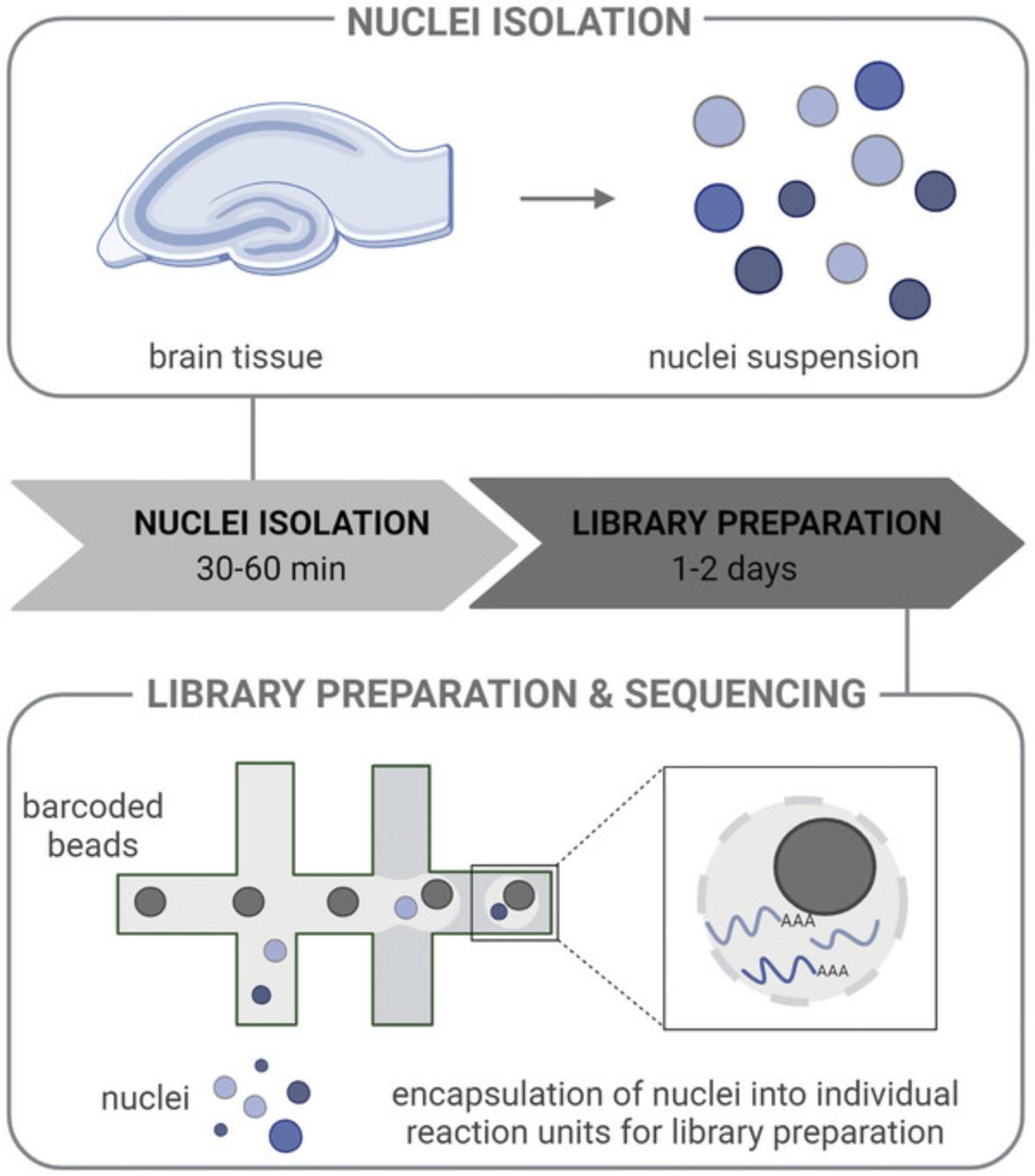
STRATEGIC PLANNING
The advantages of single-cell data come at very high costs compared to conventional bulk sequencing. These costs mainly arise from the need to use specialized equipment or performing individual reactions per cell. Therefore, it is crucial to critically determine whether the experiment requires a single-cell readout, whether cells or nuclei should be used, and which platform should be chosen to answer the biological question. Additionally, the timing, cost-benefit consideration, and experimental design need to be considered before the experiment.
Bulk versus Single-cell Readouts
A cell type-specific transcriptional readout can be obtained without single-cell sequencing if the specific cell type of interest is known. Different options to enrich certain cell types exist, e.g., Fluorescence Activated Cell Sorting (FACS), which sorts fluorescently labeled cells, or nuclei, of specific cell populations, e.g., neural and non-neural cells (Dammer et al., 2013; Haenni et al., 2012). Bulk sequencing approaches can then be performed on these cells/nuclei, thus avoiding expensive single-cell sequencing. This approach is compatible with our nuclei dissociation protocol but requires either the use of a transgenic mouse line expressing a fluorophore under a cell type-specific promoter, or a cell type-specific marker protein that can be used to stain the nuclei sample in a first step. In this case, a proper marker needs to be identified that is present within the nucleus. Alternatively, other techniques exist that provide cell type-specific bulk RNA readouts, e.g., translating ribosome affinity purification (TRAP) (Heiman et al., 2014), which captures ribosome-bound RNAs and thus the translatome, and do not require the preparation of a single-nuclei suspension.
Single-cell versus Single-nucleus Sequencing
As discussed in the introduction, snRNA-seq provides several advantages. However, in cases where a readout of mRNAs within the whole cell is necessary, e.g., because of the localization of transcripts, a single-cell protocol should be chosen instead.
Sequencing Platforms
Amongst the different platforms for snRNA-seq, Smart-seq2 and 10x Genomics (Wang et al., 2021) are currently the most popular ones. Smart-seq2 offers the advantage of full-length transcript coverage (Picelli et al., 2014). However, library preparation is performed per cell and in a plate, thus requiring initial sorting, e.g., with FACS. This results in high costs per cell as only a few hundred cells are typically processed. In comparison, 10x Genomics is a droplet-based technique and requires a specialized device that allows encapsulation of single cells or nuclei with barcoded beads in individual reaction units within the sample (Fig. 1) (Zheng et al., 2017). One library can be prepared from each sample instead of each individual cell, thereby lowering the cost per individual cell. 10x Genomics allows the processing of thousands of cells at once, enabling the detection of a broad range of cell types and even rare cell populations. In comparison, Smart-seq usually detects more genes per cell, therefore, it is better suited for lowly expressed genes (Wang et al., 2021). Both techniques can be performed with single cells or nuclei. Additionally, once a single-nuclei suspension has been generated, cost-effective protocols exist that circumvent the use of specialized equipment or multiple reactions, such as PIP-seq (Clark et al., 2023) or SPLiT-seq (Rosenberg et al., 2018).
Timing
To ensure high quality single-cell transcriptional data, the time between the preparation of the nuclei and loading the chromium controller should be minimized. Once extracted, the nuclei cannot be preserved and should not be frozen, thus sample preparation should be performed close to a chromium controller, which is usually located at a core facility. The protocol for the preparation of a single-nucleus suspension described here is comparatively fast (about 30 min) and only requires a bench-top centrifuge, thus allowing the protocol to be flexibly performed in standard laboratories.
Cost-benefit Considerations
The experimental cost is strongly influenced by the number of samples that are analyzed, and by the number of nuclei that are profiled per sample. A higher number of nuclei will increase the multiplet rate, thus impeding proper cell type allocation. Typically, doublets formed by nuclei of distinct transcriptional states are therefore removed during the analysis. Therefore, the decision on how many nuclei will be profiled is a trade-off between the potential capture of sparser cell types and the multiplet rate.
Experimental Design
Due to the high costs of single-cell sequencing, group sizes are usually kept small. Nevertheless, it is essential to analyze multiple samples (with each sample constituting one biological replicate), ideally three or more per group, to make quantitative statements and allow meaningful interpretation of the results. Ideally, group size should be based on a power calculation, with larger effect sizes requiring fewer biological replicates. Any experiment with only one sample per group will not be interpretable due to the high variability between samples. Importantly, different cells are not experimentally independent, and as such do not constitute replicates (Lazic, 2010; Squair et al., 2021). To differentiate biological treatment effects from those introduced by confounding variables, the experimental design needs to be properly planned beforehand (for planning advice, consult Baran-Gale et al., 2018; Dal Molin & Di Camillo, 2018; Nguyen et al., 2018). Confounding factors can be introduced by the experimenters, by the chromium chip used to run the samples, or by the sequencing lanes. Ideally, all samples are processed using a block-design, with each block containing one sample of each group (Auer & Doerge, 2010) (Fig. 2). Within each block, the samples are processed in a randomized order. This order can be maintained throughout the experimental process, from sample preparation to sequencing all samples in the same lane. In case multiple experimenters are involved in the nuclei preparation, this confounding factor should also be accounted for.
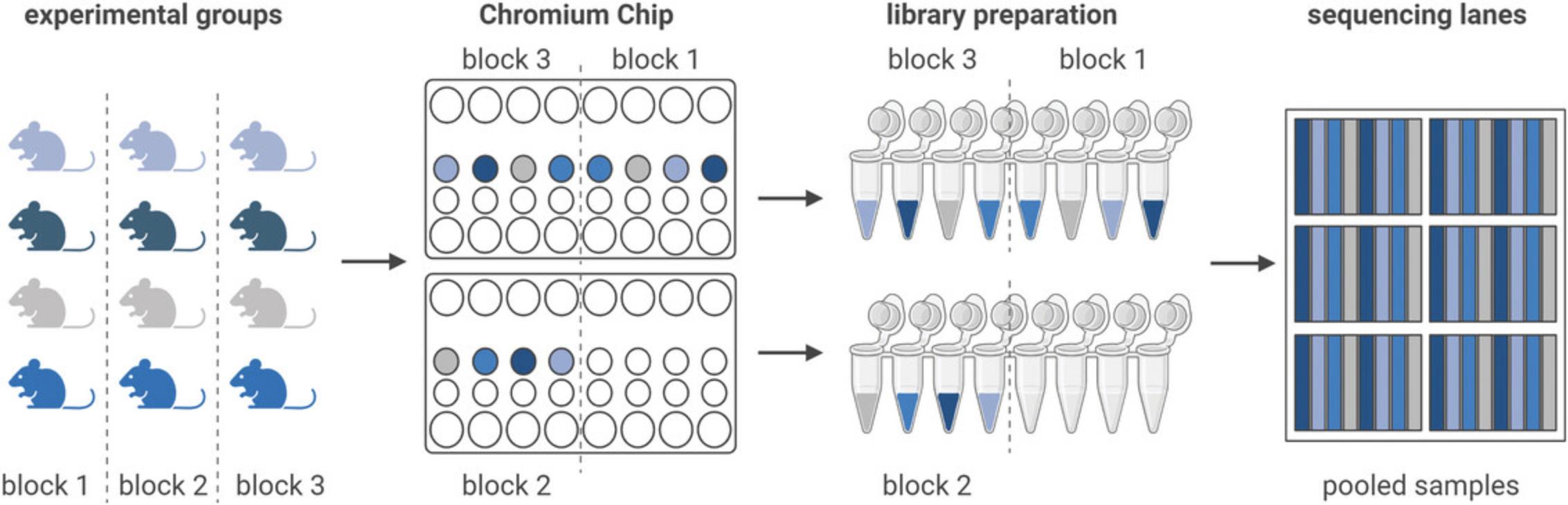
NOTE: All protocols involving animals must be reviewed and approved by the appropriate Animal Care and Use Committee and must follow regulations for the care and use of laboratory animals. Appropriate informed consent is necessary for obtaining and use of human study material.
Basic Protocol 1: PREPARATION OF SINGLE-NUCLEUS SUSPENSION
Here, we provide an in-depth protocol for the generation of a single-nucleus suspension from flash-frozen brain tissue (Fig. 3A). This protocol relies on the mechanical disruption of tissue in a lysis buffer. The nuclei are further cleaned by centrifugation and filtering. Although we use hippocampal tissue (approximately 15 to 25 mg per sample), this protocol should be applicable for a wide variety of brain regions.
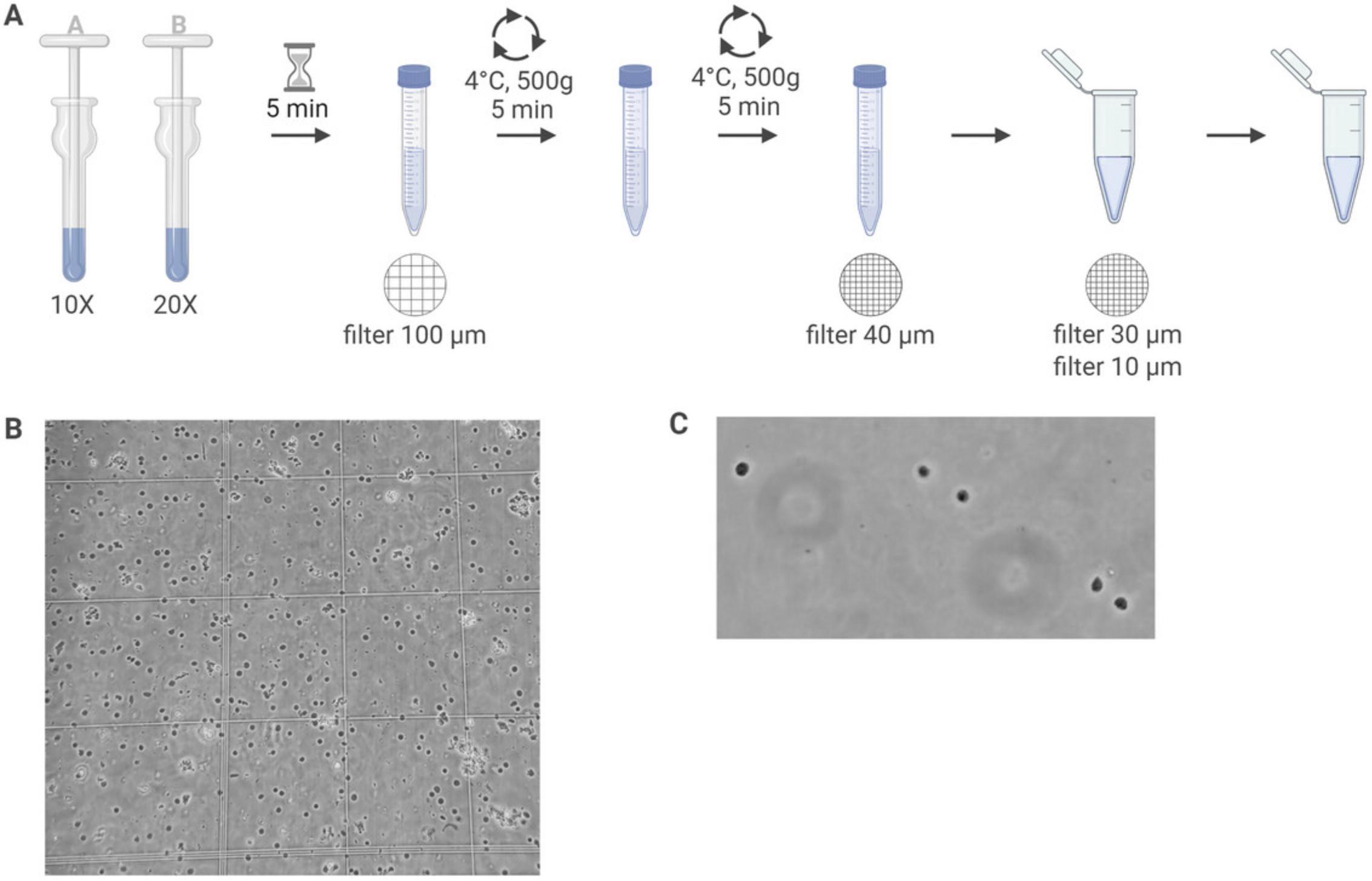
For successful nuclei isolation, it is critical to keep the tissue frozen on dry ice or in liquid nitrogen until the mechanical disruption. The success of this protocol can be evaluated by counting the nuclei and assessing their quality (Fig. 3B). A nucleus of good quality shows an intact membrane and is round in shape. Over-lysed nuclei will show signs of leakiness and blebbing. As the nuclei contain no rRNA, no quality control for RNA integrity can be obtained after nuclei isolation. In case this is required, a standard cDNA conversion can be performed directly on the nuclei before moving to the single-cell readout. However, in our experience this is not necessary if RNase inhibitors were used and the nuclei seem intact.
Materials
- RNase away
- Double-distilled water (ddH2O)
- Input tissue (flash-frozen, -80°C)
- Lysis buffer (see recipe)
- Wash buffer (see recipe)
- 0.4% Trypan blue (Millipore Sigma, cat. no. T8154, or equivalent)
-
- Dounce homogenizer (Millipore Sigma, cat. no. D9063)
- Beaker, large
- Aluminum foil
- Centrifuge, fixed-angle or swing-bucket
- CellTrics strainer, 100-µm (Sysmex, cat. no. 04-004-2328)
- 50-ml Conical tubes
- 15-ml Conical tubes
- 1.5-ml Microcentrifuge tubes
- Flowmi cell strainer, 40-µm (Millipore Sigma, cat. no. BAH136800040)
- CellTrics strainer, 30-µm (Sysmex, cat. no. 04-004-2326)
- CellTrics strainer, 10-µm (Sysmex, cat. no. 04-004-2324)
- Counting chambers (Carl Roth, cat. no. PK36.1)
- Brightfield microscope
Preparation
NOTE: All steps need to be performed on ice.
1.Clean all surfaces with RNase away.
2.Prepare buffers following the recipes as they appear in the Reagents and Solutions section.
3.Rinse Dounce homogenizer and pestle with ddH2O before use, wrap in aluminum foil, and store in the refrigerator at 4°C until use.
4.Prepare a large beaker with ddH2O for cleaning the Dounce homogenizer and pestles.
5.Cool down centrifuge to 4°C.
6.Add RNase inhibitors to the lysis buffer directly before starting the protocol (see recipe).
Nuclei isolation
7.Add 1 ml of cold lysis buffer to the Dounce homogenizer and then add your tissue. Make sure the tissue is fully submerged in the lysis buffer.
8.Homogenize the tissue with 10 strokes of pestle A (loose pestle).
9.Homogenize the tissue with 20 strokes of pestle B (tight pestle).
10.Add 2 ml of lysis buffer to the Dounce homogenizer.
11.Incubate on ice for 5 min.
12.Add 4 ml wash buffer to the Dounce homogenizer.
13.Pour homogenate through a 100-µm CellTrics filter into a fresh 50-ml conical tube.
14.Transfer supernatant into a 15-ml conical tube and add 5 ml wash buffer.
15.Centrifuge 5 min at 500 × g , 4°C.
16.Discard supernatant.
17.Add 1 ml wash buffer and resuspend the pellet using a P1000.
18.Transfer the liquid to a new 15-ml conical tube.
19.Add 4 ml wash buffer and fully resuspend the pellet using a P1000.
20.Centrifuge for 5 min at 500 × g , 4°C.
21.Discard supernatant.
22.Add 1 ml wash buffer and fully resuspend the pellet using a P1000.
23.Aspirate all liquid using a P1000 tip, attach a Flowmi 40-µm filter and slowly release the suspension through the filter into a 1.5-ml microcentrifuge tube.
24.Filter solution through a 30-µm CellTrics filter into a new tube.
25.Filter solution through a 10-µm CellTrics filter into a new tube.
Counting of nuclei
26.Directly before counting, mix the nuclei suspension very well by carefully pipetting with a P1000 pipet.
27.Transfer 10 µl of nuclei suspension/dilution into a new tube and add 10 µl of Trypan blue. Mix carefully by pipetting and wait 2 minutes.
28.Add 10 µl of the mix to a counting chamber.
29.Count nuclei from all four squares and calculate the concentration of your nuclei suspension.
30.Assess nuclei quality of the suspension using a brightfield microscope. Intact nuclei are round in shape and can be easily differentiated from debris and ruptured nuclei (Fig. 3C).
Cleaning
31.Properly clean the Dounce homogenizer and pestle under water until there is no foam or tissue pieces left. Place them for drying and rinse again with ddH2O before further use.
32.Buffers can be kept at 4°C and used within 24 hr.
Basic Protocol 2: PREPARATION AND SEQUENCING OF SINGLE-NUCLEUS LIBRARIES FOR RNA-SEQ
Single-nucleus RNA-seq using the 10x Genomics assay depends on the generation of microdroplets, called gel beads in emulsion (GEMs). Each GEM contains, ideally, one barcoded gel bead and one nucleus. Within these individual reaction units, cDNA synthesis is performed to allow the tracing of multiple reads back to a single nucleus (Fig. 4A).
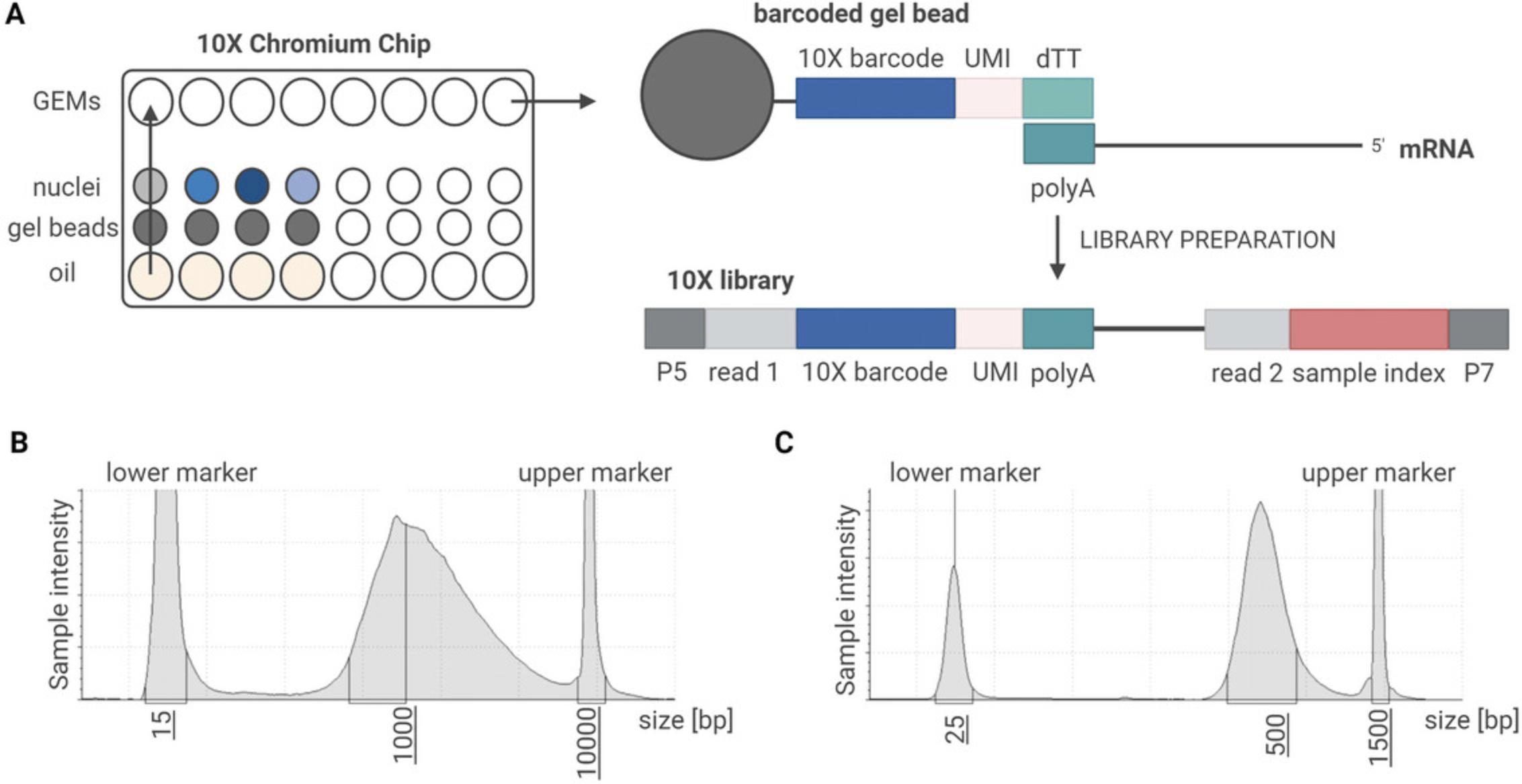
We performed cDNA synthesis and library preparation following the chromium single-cell 3’ reagent kit v3 user guide (CG000183 Rev B). Each version of the chromium single-cell 3’ reagent kit v3 user guide contains detailed instructions on how to prepare each individual reaction mix. However, the general steps are similar to newer versions of the RNA-seq protocol provided by 10x Genomics.
Materials
- Single-nucleus suspension (see Basic Protocol 1)
- Chromium single-cell 3ʹ gel bead kit v3 (10x Genomics, cat. no. PN-1000076)
- Chromium single-cell 3ʹ GEM kit v3 (10x Genomics, cat. no. PN-1000077)
- Dynabeads™ MyOne™ SILANE (10x Genomics, cat. no. PN-2000048)
- 80% Ethanol
- SPRIselect reagent kit (Beckman Coulter, cat. no. B23318)
- Chromium single-cell 3ʹ library kit v3 (10x Genomics, cat. no. PN-1000078)
- Chromium i7 multiplex kit (10x Genomics, cat. no. PN-220103)
- Buffer EB (Qiagen, cat. no. 19086)
- Kapa DNA quantification kit (Roche, cat. no. KK4824)
- 50% Glycerol (Ricca Chemical Company, cat. no. 3290-32)
-
- 10x Genomics chromium controller
- 10x Genomics chromium accessories
- 10x Vortex adapter (cat. no. 330002)
- 10x Vortex clip (cat. no. 230002)
- 10x Chip holder (cat. no. 330019)
- 10x Magnetic separator (cat. no. 230003)
- Thermal cycler
- Agilent 4200 tapestation system/bioanalyzer 2100
- Sequencing system
Preparation
1.Count nuclei and calculate the concentration of nuclei as previously described (see Basic Protocol 1).
2.Turn on the chromium controller.
Compartmentalized reverse-transcription
3.Thaw all reagents for the reverse-transcription, vortex the gel beads thoroughly.
4.Dilute nuclei in water according to the calculated nuclei concentration, aiming for your desired nuclei concentration.
5.Assemble the chromium chip as shown in the user guide.
6.Prepare the master mix containing all reagents required for the reverse transcription on ice.
7.Mix the master mix and the nuclei dilutions on ice.
8.Load the chromium chip by adding the requested volume of nuclei suspension, gel beads, and partitioning oil. Fill all unused wells with 50% glycerol solution.
9.Immediately close the chip and start the chromium run.
10.After the run is finished, slowly aspirate 100 µl of the formed GEMs from the first row of the chip.
11.Transfer 100 µl of the GEMs into a pre-cooled 0.2-ml 8-strip tubes on ice.
12.Incubate the sample in the thermal cycler and immediately start reverse-transcription (lid temperature 53°C).
45 min | 53°C |
5 min | 85 °C |
Hold | 4°C |
cDNA amplification
13.Thaw all reagents for the amplification.
14.Remove the sample from the thermal cycler and immediately add recovery agent to break all GEMs and release cDNA.
15.Perform a clean-up using Dynabeads.
16.Recover cDNA in elution solution I.
17.Prepare the amplification mix on ice.
18.Mix the amplification mix and the cDNA.
19.Incubate the sample in the thermal cycler for cDNA amplification to generate sufficient amounts of cDNA while minimizing amplification biases (lid temperature 105°C).
1 cycle | 3 min | 98°C |
n cycles | 15 sec | 98°C |
20 sec | 63°C | |
1 min | 72°C | |
1 cycle | 1 min | 72°C |
Hold | 4°C |
20.Perform a SPRIselect clean-up.
21.Perform two washes with 80% ethanol by pipetting on the other side of the tube to not disturb the beads. Recover cDNA in EB buffer.
22.Perform quality control (QC) using Bioanalyzer or Tapestation (Fig. 4B).
Library preparation
23.Thaw all reagents for the fragmentation and ligation step.
24.Prepare fragmentation mix on ice.
25.Mix a fraction of the amplified cDNA with the fragmentation mix and incubate on the thermal cycler (lid temperature 65°C). Immediately start the fragmentation (32°C) once the samples are in the pre-cooled thermal cycler.
Pre-cool | 4°C |
5 min | 32°C |
30 min | 65°C |
Hold | 4°C |
26.Perform a double-sided SPRI size selection.
27.Recover cDNA in EB buffer.
28.Prepare adaptor ligation mix.
29.Mix fragments with the adaptor ligation mix and incubate on a thermal cycler (lid temperature 30°C).
15 min | 20°C |
Hold | 4°C |
30.Perform a clean-up using SPRI size selection to select fragments in the range of 200 to 600 bp.
31.Recover the fragments in EB buffer.
32.Prepare sample index PCR mix.
33.Mix the cleaned fragments with the samples index PCR mix and the indexes.
34.Incubate on a thermal cycler (lid temperature 105°C).
1 cycle | 45 min | 98°C |
n cycles | 20 sec | 98°C |
30 sec | 54°C | |
20 sec | 72°C | |
1 cycle | 1 min | 72°C |
Hold | 4°C |
35.Perform double-sided SPRI size selection to select fragments in the range of 200 to 600 bp.
36.Recover fragments in EB buffer.
37.Perform QC using Bioanalyzer or Tapestation (Fig. 4C).
Sequencing
38.Calculate the molarity of each sample and pool the libraries that will be sequenced together accordingly.
39.Sequence the pool using the following recommended parameters on an Illumina sequencing platform:
- Read 1: 28 bp
- Read 2: 91 bp
- i7 index read: 8 bp
REAGENTS AND SOLUTIONS
Reagents should be prepared under clean and RNase-free conditions. Prepared solutions should be kept at 4°C and used on the day of preparation.
BSA, 5% (10 ml)
- 500 mg BSA powder (Jackson ImmunoResearch, cat. no. 001-000-162)
- PBS pH 7.4 (ThermoFisher, cat. no. 10010023)
Fill up to 10 ml with PBS. Invert tube and keep at 4°C until fully dissolved. To prevent bubbles, do not shake. Filter sterilize solution before use.
Lysis buffer (10 ml)
- 9.700 ml ddH2O
- 20 μl 5M NaCl (MilliporeSigma, cat. no. 59222C)
- 100 μl 10% NP-40 (Abcam, cat. no. ab142227)
- 30 μl 1M MgCl2 (ThermoFisher, cat. no. AM9530G)
- 100 μl 1M Tris·HCl (Sigma, cat. no. T2194)
- 50 μl Protector RNase inhibitor (MilliporeSigma, cat. no. 3335399001)
Keep the RNase inhibitor at -20°C; add directly before use.
Wash buffer (50 ml)
- 10 ml 5% BSA
- 39.75 ml PBS pH 7.4 (ThermoFisher, cat. no. 10010023)
- 250 μl Protector RNase inhibitor (MilliporeSigma, cat. no. 3335399001)
Keep the RNase inhibitor at -20°C; add directly before use.
COMMENTARY
Background Information
Since the first high-throughput sequencing of a single cell (Tang et al., 2009), the field has rapidly evolved and increased in popularity. While initially, single-cell sequencing was aimed at profiling very rare cell types that contain only limited transcriptional material, once applied to multiple cells, it became clear that the transcriptional information obtained not only provides information about treatment effects, but also allows the separation of individual cell types (Svensson et al., 2018). Since then, single-cell sequencing approaches have been used for multiple reasons, e.g., to identify (novel) cell types and quantify their abundance, to profile very rare cell types, and to compare treatment effects on a single-cell and/or pseudo-bulk level.
We have used this protocol to measure the transcriptional impact of acute stress exposure on the adult mouse hippocampus, a stress-sensitive brain region (von Ziegler et al., 2022). Many hippocampal single-cell sequencing datasets exist (Chang et al., 2023; Zeisel et al., 2015), the cell types of the hippocampus are very well characterized, and individual cell types even show distinct transcriptional signatures based on their distribution along the longitudinal axis of the hippocampus (Cembrowski et al., 2016). As we are interested in stress-induced regulation of immediate-early genes, which are easily biased by the dissociation technique, we performed snRNA-seq. Because snRNA-seq focuses on nascent transcripts, and due to the depletion of cytosolic and more mature mRNAs, it captures actively ongoing nuclear transcription better than scRNA-seq. Therefore, we were able to show strong stress-induced changes in active transcription (von Ziegler et al., 2022). The identified genes corresponded with those identified in bulk RNA-seq, and several of them could be exclusively attributed to changes in one specific cell type (e.g., upregulation of Apold1 in vascular cells). Overall, we found that most of the stress-induced gene expression changes occurred in glial cells, specifically in astrocytes.
Critical Parameters
When preparing samples for snRNA-seq, working clean and on ice is critical for all parts of the protocol. In addition, several parameters of this protocol were found to work well with hippocampal tissue but allow for some adjustments if other tissues need to be profiled.
RNA integrity
To obtain samples with high RNA integrity, it is of utmost importance to work under clean conditions and use RNase inhibitors during the sample preparation. Equally, all solutions should be freshly prepared and kept clean. While usually high amounts of RNase inhibitors are recommended, using 0.2 U/µl has proven sufficient in our lab for maintaining high quality RNA when preparing the nuclei suspension, which greatly helps to reduce cost.
Input tissue
In our experience, the protocol works well for 5 to 20 mg of brain tissue. If a similar amount of tissue is used, the protocol can be followed without alterations. If more, or less, tissue is used the volumes may need to be adjusted. For a small amount of input tissue, some steps can potentially be omitted as outlined below to minimize nuclei loss. However, such variations need to be tested specifically for each tissue or tissue amount used.
Timing
Due to the design of the chromium chip, a maximum of 8 samples can be prepared for single-nucleus sequencing in one round of sample preparation with one chip. These samples can be easily handled by one person for library preparation. For the preparation of nuclei, the time the sample is exposed to the lysis buffer is critical. Therefore, depending on the number of samples that should be processed, it can be necessary to prepare samples as a team. In this case, it is critical to account for the different experimenters, which represent confounding variables, in the later analysis and the experimental design needs to be adjusted accordingly (see Experimental Design in Strategic Planning section and Fig. 2).
Debris Removal
Single-nucleus sequencing using the 10x Genomics assays relies on the use of the chromium controller, a device that performs single nucleus partitioning. The process of separating the nuclei into GEMs, each containing (ideally) one gel bead and one nucleus, enables the single-nucleus readout. For the successful generation of GEMs, the sizes of the cells or nuclei is important. In the case of single-nucleus sequencing, different nuclei do not vary strongly in size, therefore, this can be neglected. However, as a single-nucleus suspension is obtained from a frozen tissue sample, the suspension will contain a large amount of debris. To remove larger particles, this protocol uses filters with different mesh sizes. In this protocol, we start by using filters of higher mesh sizes, to first remove larger pieces of tissue and then titrate down to very narrow mesh sizes. While the filter sizes outlined in this protocol worked well for hippocampal tissue, additional filtering steps can be added if needed based on visual inspection. For this, we recommend visually inspecting the single-nucleus suspension at various points throughout the preparation to observe if more, or less, filtering steps need to be applied. It is important to consider the trade-off between debris removal with filters and the inherent loss of nuclei that comes with each filtering step.
It is very difficult to fully clean a single-nucleus suspension. In our experience, this is also not required for a successful single-nucleus sequencing project. A certain amount of debris is tolerable and will not interfere with the sequencing, as long as the remaining particles are smaller than the nuclei and do not form large aggregates that can potentially clog the chromium chip. If a large amount of debris remains present in the sample even after filtering with small sized filters, a density gradient centrifugation can be performed (Nott et al., 2021). In this case, all other filtering steps can be omitted. In our experience, density gradient centrifugation greatly helps to remove debris, but leads to the loss of high numbers of nuclei and greatly prolongs the sample preparation steps. Therefore, it is not included in this protocol.
Centrifugation
The mechanical disruption of frozen tissue into single nuclei will release high amounts of cytosolic RNAs, as well as RNAs from broken nuclei, into the suspension. If these fragments are not removed, they will appear as background in all GEMs, as they will get barcoded together with mRNAs from the nuclei within the GEM. This can massively blur the sequencing results and impede proper cell type annotation. Therefore, removal of such fragments during nuclei isolation is critical. Several centrifugation steps are applied to exchange the suspension while keeping the nuclei pellet. Both fixed-angle and swing-bucket centrifuges work well for this protocol. In the case of very low nuclei recovery, using a swing-bucket centrifuge may be more suitable as they allow for easier retention of the nuclei pellet.
Troubleshooting
There are several potential pitfalls in the preparation of samples for snRNA-seq that can be addressed by visual inspection of the nuclei suspension. Troubleshooting advice can be found in Table 1.
Problem | Possible cause | Solution |
---|---|---|
Low nuclei yield | Amount of input material insufficient | Calculate nuclei concentration directly after homogenization, if already too low, increase amount of input material by pooling tissue from multiple animals |
Centrifugation and filtering leads to high loss of nuclei | Calculate nuclei concentrations at several steps of the protocol and test whether one or several of the filtering steps can be omitted without negatively impacting the amount of debris. Alternatively, use more input material | |
Poor nuclei quality | Mechanical disruption too harsh | Repeat protocol with less strokes, nuclei can be captured after several strokes to see how their quality decreases with additional strokes |
Lysis buffer too strong | Try different concentrations of NP-40 and compare nuclei quality between protocols | |
Large amount of debris | Debris is not sufficiently removed | Add additional filtering steps, or a density gradient step can be added but it will increase the time needed for sample preparation. A FACS step can be added to the protocol which will strongly reduce the amount of debris. We did not find this necessary for our experiments and it introduces long time delays and additional artifacts |
Low RNA integrity | Insufficient RNase inhibitor used | We have found 0.2 U/μl RNase inhibitor to be suggicient but the amount can be increased up to 2 U/μl (this will increase cost) |
Statistical Analysis
Single-cell libraries prepared using a 10x Genomics assay include three sequences that allow for de-multiplexing at different steps of the library preparation (Fig. 5). First, each read contains a unique molecular identifier (UMI) sequence. This sequence is introduced during cDNA conversion once the nuclear mRNA binds to the barcoded bead (Fig. 4A). As the preparation of single-nucleus libraries consists of multiple amplification steps, the use of UMIs allows to trace every count back to the individual RNA fragment it originated from, thus allowing quantitative analyses. Additionally, during cDNA conversion, a 10x barcode is introduced to each individual sequence. While the UMI is different for each mRNA fragment to which a barcoded bead will bind, the 10x barcode is the same for all fragments within one specific GEM, thus allowing to trace a group of reads with the same 10x barcode back to one nucleus. At a later step, a sample index is introduced to the library. This sequence is unique for every sample and thus allows pooling different samples for sequencing.
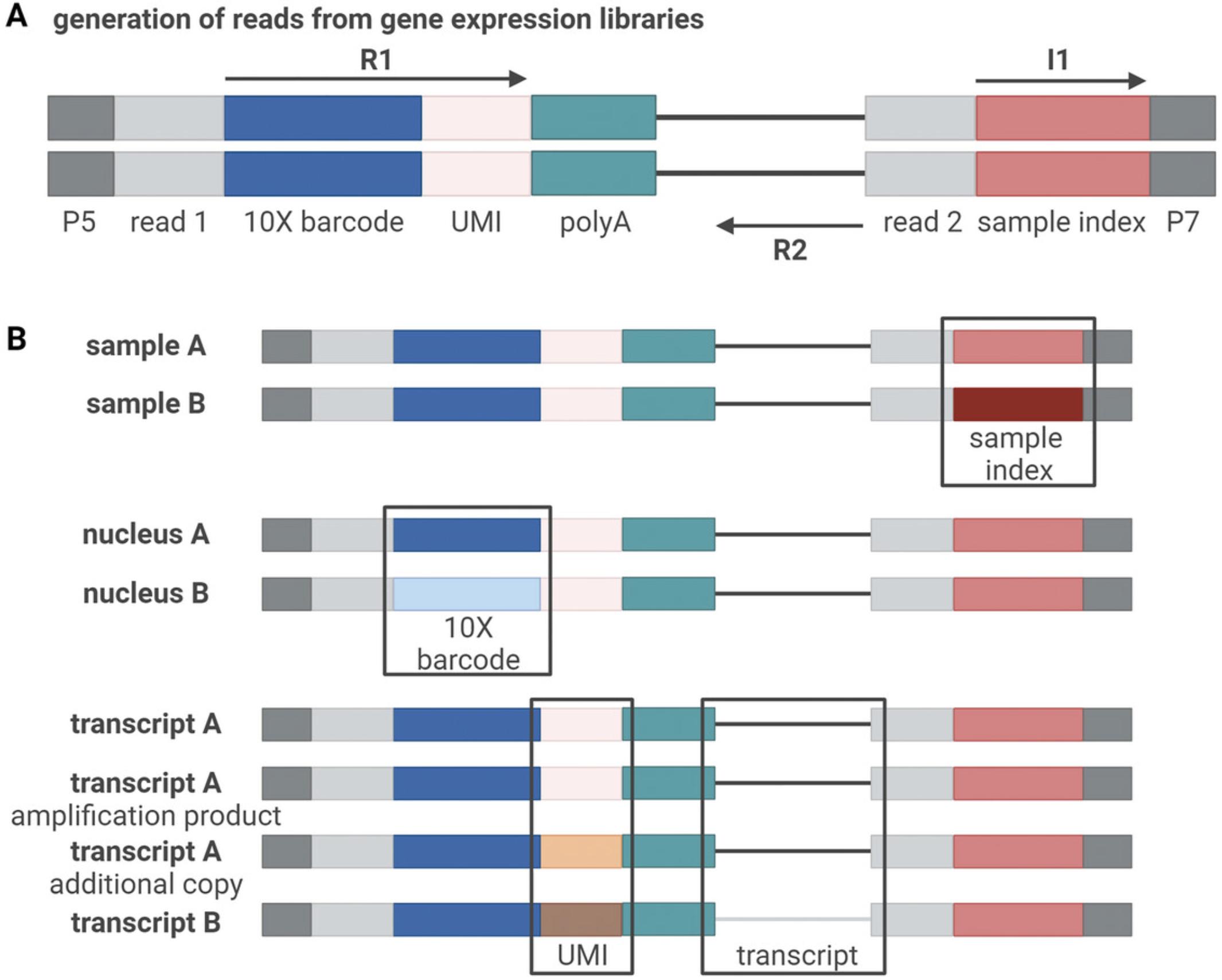
Once sequencing has been performed and the data have been de-multiplexed based on the sample index, the data are returned in the FASTQ format. Typically, three FASTQ files are generated for each sample (four if dual indexing was used), with one file containing the sample index (I1), one containing the 10x barcode and UMI (R1), and one containing the transcript read (R2) (Fig. 5A). Therefore, despite single-cell libraries usually being sequenced paired-end, the reads are mapped to the reference genome like single-end data.
The FASTQ files can be processed with CellRanger (Zheng et al., 2017), which will also provide basic quality measures, although less computationally-intensive alternatives also exist (He et al., 2022). A step-by-step explanation for CellRanger can be found on the 10x webpage (https://support.10xgenomics.com/single-cell-gene-expression/software/pipelines/latest/using/tutorials). The CellRanger pipeline performs four distinct steps: 1) assign reads to droplets, 2) align those reads to the reference genome, 3) quantify the UMIs per transcript per droplet and 4) call which droplets contain cells. For alignment, pre-built reference genomes can be downloaded from the 10x website for human and mouse. As the majority of transcripts in snRNA-seq are unspliced (i.e., including introns), it is common to use unspliced transcripts, or both spliced and unspliced transcripts, for UMI counting. For other organisms, custom references need to be designed. After completing the CellRanger processing, a report is obtained for each sample that summarizes important information about the quality of the sequencing, including the number of cells obtained, the number of reads per cell, the percentage of mapped reads and additional information for each sample. Further, CellRanger outputs two feature-barcode matrices, one raw matrix containing data from all droplets (including empty droplets that can be used for background subtraction), and one filtered feature-barcode matrix containing the counts per gene for all cells that were called. However, further quality filtering is generally necessary. Cells with low library size or number of detected genes, or with a large fraction of the reads assigned to a very small fraction of transcripts, should be excluded. Moreover, although snRNA-seq should in principle not contain mitochondrial reads, in practice the cells generally do in small amounts, and cells with a high proportion should be excluded. While no optimal thresholds have been established for such filtering, it is commonly done using deviation from the distribution across cells and should not be too stringent to avoid bias against certain cell types (Germain et al., 2020). Finally, doublets ought to be removed using scDblFinder (Germain et al., 2022).
For data analysis, Seurat (Stuart et al., 2019) and the Bioconductor framework (Amezquita et al., 2020) are popular and very well-documented choices, which can work with the filtered feature-barcode matrix. Both allow users to filter the reads, apply normalizations and scaling for different dimensionality reductions, and perform differential expression analyses. If required, ambient RNA contaminations, present in all droplets (also empty ones), can be removed using CellBender beforehand (Fleming et al., 2023). To avoid spurious results, differential expression analysis across conditions is best performed at the pseudo-bulk level (Crowell et al., 2020; Squair et al., 2021). As the tools to analyze single-cell sequencing data evolve very rapidly, analysis techniques are not standardized, and a number of analysis challenges represent active research topics (Lähnemann et al., 2020; Stegle et al., 2015).
Understanding Results
While pipelines exist that can readily compute differentially expressed genes in single-cell data, the functional interpretation of these results remains complex. Despite the great advantages of snRNA-seq and scRNA-seq using the 10x Genomics assay, it suffers from a lower capture efficiency, with only a fraction of transcripts actually being sequenced per cell (Ding et al., 2020). This results in sparse data compared to bulk readouts. Therefore, great caution is warranted when any conclusions are drawn about the lack of certain transcripts in scRNA-seq and snRNA-seq. Additionally, only a subset of all cells is sequenced, typically up to 10,000 cells (due to the increasing number of doublets) using the 10x Genomics single-cell gene expression assay. Therefore, differences in the sequencing results of single-cell techniques compared to bulk RNA-seq are common and expected. When directly comparing snRNA-seq, which captures active transcription, with bulk RNA-seq data, the bulk data should be reduced to the unspliced reads. In snRNA-seq, the readout is additionally narrowed to the nuclear compartment, and the absence of non-nuclear transcripts poses another restriction on comparative analyses (Lake et al., 2017). Finally, it can be difficult to detect changes in the expression of genes that quickly translocate from the nucleus to other cellular compartments, such as Arc (Lyford et al., 1995; Steward et al., 1998).
For brain tissue, single-cell technologies typically perform well in capturing common cell types. However, the abundance of each cell type is fragile and sensitive to the dissociation protocol. Additionally, droplet-based single-cell approaches seem to preferentially capture non-astrocytic cells, resulting in reduced capture rates of astrocytes (Liddelow et al., 2020). This can result in underpowered studies, as the number of cells usually compensates for the reduced sequencing depth. Insufficiently powered sample sizes can thus result in biologically inaccurate clustering or differential expression analysis. If a certain cell type is of special interest for a given experiment, certain techniques to enrich the cell type of interest should therefore be applied beforehand (e.g., FACS).
It is important to keep in mind that for many biological questions, the preparation of the sample could impact the results. Several studies have shown an upregulation of certain transcripts, especially those associated with neuronal activation, like immediate-early genes, in different tissue types (Lacar et al., 2016; van den Brink et al., 2017). However, certain cell types, such as microglia, are known to be especially susceptible to dissociation artifacts, thus dissociation-induced activation of IEGs could mask the transcriptional changes induced by neuronal activation, specifically in microglia (Marsh et al., 2022). While there is an increasing number of publications that evaluate such dissociation artifacts and propose optimized protocols, there are still many unknown sources of biases. To minimize artifacts, our protocol was designed to avoid both heat exposure and long processing times, and it uses filtering instead of cell sorting to remove debris. However, unknown sources of artifacts remain and necessitate careful interpretation of snRNA-seq data.
Time Considerations
For a trained user, the preparation of a single-nucleus suspension takes between 30 to 60 min, depending on the number of samples and experimenters (Fig. 1). The library preparation protocol can be finished within 1 to 2 days, additional time is required for sequencing.
Acknowledgments
The authors thank Ferdinand von Meyenn and Laura Hinte for help setting up single-nucleus sequencing and Pierre-Luc Germain for critical reading and thoughtful comments on the manuscript. The lab of J.B. is supported by the ETH Zurich, ETH Project Grant ETH-20 19-1, SNSF Grants 310030_172889 and 310030_204372, the Botnar Research Centre for Child Health, the Swiss 3R Competence Center, Roche, and the Hochschulmedizin Zürich Flagship project STRESS. Figures were created with BioRender.com.
Author Contributions
Rebecca Waag : Conceptualization; methodology; visualization; writing—original draft; writing—review and editing. Johannes Bohacek : Conceptualization; funding acquisition; resources; writing—review and editing.
Conflict of Interest
The authors declare no conflict of interest.
Open Research
Data Availability Statement
Data sharing not applicable to this article as no datasets were generated or analyzed during the current study.
Literature Cited
- Adam, M., Potter, A. S., & Potter, S. S. (2017). Psychrophilic proteases dramatically reduce single-cell RNA-seq artifacts: A molecular atlas of kidney development. Development , 144(19), 3625–3632. https://doi.org/10.1242/DEV.151142
- Amezquita, R. A., Lun, A. T. L., Becht, E., Carey, V. J., Carpp, L. N., Geistlinger, L., Marini, F., Rue-Albrecht, K., Risso, D., Soneson, C., Waldron, L., Pagès, H., Smith, M. L., Huber, W., Morgan, M., Gottardo, R., & Hicks, S. C. (2020). Orchestrating single-cell analysis with Bioconductor. Nature Methods , 17(2), 137–145. https://doi.org/10.1038/s41592-019-0654-x
- Auer, P. L., & Doerge, R. W. (2010). Statistical design and analysis of RNA sequencing data. Genetics , 185(2), 405–416. https://doi.org/10.1534/genetics.110.114983
- Bakken, T. E., Hodge, R. D., Miller, J. A., Yao, Z., Nguyen, T. N., Aevermann, B., Barkan, E., Bertagnolli, D., Casper, T., Dee, N., Garren, E., Goldy, J., Graybuck, L. T., Kroll, M., Lasken, R. S., Lathia, K., Parry, S., Rimorin, C., Scheuermann, R. H., … Tasic, B. (2018). Single-nucleus and single-cell transcriptomes compared in matched cortical cell types. PloS One , 13(12), e0209648. https://doi.org/10.1371/JOURNAL.PONE.0209648
- Baran-Gale, J., Chandra, T., & Kirschner, K. (2018). Experimental design for single-cell RNA sequencing. Briefings in Functional Genomics , 17(4), 233–239. https://doi.org/10.1093/bfgp/elx035
- Buenrostro, J. D., Giresi, P. G., Zaba, L. C., Chang, H. Y., & Greenleaf, W. J. (2013). Transposition of native chromatin for fast and sensitive epigenomic profiling of open chromatin, DNA-binding proteins and nucleosome position. Nature Methods , 10(12), 1213–1218. https://doi.org/10.1038/nmeth.2688
- Cembrowski, M. S., Wang, L., Sugino, K., Shields, B. C., & Spruston, N. (2016). Hipposeq: a comprehensive RNA-seq database of gene expression in hippocampal principal neurons. eLife , 5, e14997. https://doi.org/10.7554/eLife.14997
- Chang, C., Zuo, H., & Li, Y. (2023). Recent advances in deciphering hippocampus complexity using single-cell transcriptomics. Neurobiology of Disease , 179, 106062. https://doi.org/10.1016/j.nbd.2023.106062
- Clark, I. C., Fontanez, K. M., Meltzer, R. H., Xue, Y., Hayford, C., May-Zhang, A., D'Amato, C., Osman, A., Zhang, J. Q., Hettige, P., Ishibashi, J. S. A., Delley, C. L., Weisgerber, D. W., Replogle, J. M., Jost, M., Phong, K. T., Kennedy, V. E., Peretz, C. A. C., Kim, E. A., … Abate, A. R. (2023). Microfluidics-free single-cell genomics with templated emulsification. Nature Biotechnology , https://doi.org/10.1038/s41587-023-01685-z
- Crowell, H. L., Soneson, C., Germain, P.-L., Calini, D., Collin, L., Raposo, C., Malhotra, D., & Robinson, M. D. (2020). Muscat detects subpopulation-specific state transitions from multi-sample multi-condition single-cell transcriptomics data. Nature Communications , 11(1), 6077. https://doi.org/10.1038/s41467-020-19894-4
- Cuevas-Diaz Duran, R., González-Orozco, J. C., Velasco, I., & Wu, J. Q. (2022). Single-cell and single-nuclei RNA sequencing as powerful tools to decipher cellular heterogeneity and dysregulation in neurodegenerative diseases. Frontiers in Cell and Developmental Biology , 10, 884748. https://www.frontiersin.org/articles/10.3389/fcell.2022.884748
- Dal Molin, A., & Di Camillo, B. (2018). How to design a single-cell RNA-sequencing experiment: pitfalls, challenges and perspectives. Briefings in Bioinformatics , 20(4), 1384–1394. https://doi.org/10.1093/bib/bby007
- Dammer, E. B., Duong, D. M., Diner, I., Gearing, M., Feng, Y., Lah, J. J., Levey, A. I., & Seyfried, N. T. (2013). Neuron enriched nuclear proteome isolated from human brain. Journal of Proteome Research , 12(7), 3193–3206. https://doi.org/10.1021/PR400246T/SUPPL_FILE/PR400246T_SI_002.PDF
- Denisenko, E., Guo, B. B., Jones, M., Hou, R., de Kock, L., Lassmann, T., Poppe, D., Poppe, D., Clément, O., Simmons, R. K., Simmons, R. K., Lister, R., & Forrest, A. R. R. (2020). Systematic assessment of tissue dissociation and storage biases in single-cell and single-nucleus RNA-seq workflows. Genome Biology , 21(1), 130. https://doi.org/10.1186/s13059-020-02048-6
- Dimitriu, M. A., Lazar-Contes, I., Roszkowski, M., & Mansuy, I. M. (2022). Single-cell multiomics techniques: from conception to applications. Frontiers in Cell and Developmental Biology , 10, 854317. https://www.frontiersin.org/articles/10.3389/fcell.2022.854317
- Ding, J., Adiconis, X., Simmons, S. K., Kowalczyk, M. S., Hession, C. C., Marjanovic, N. D., Hughes, T. K., Wadsworth, M. H., Burks, T., Nguyen, L. T., Kwon, J. Y. H., Barak, B., Ge, W., Kedaigle, A. J., Carroll, S., Li, S., Hacohen, N., Rozenblatt-Rosen, O., Shalek, A. K., … Levin, J. Z. (2020). Systematic comparison of single-cell and single-nucleus RNA-sequencing methods. Nature Biotechnology , 38(6), 737–746. https://doi.org/10.1038/s41587-020-0465-8
- Fleming, S. J., Chaffin, M. D., Arduini, A., Akkad, A.-D., Banks, E., Marioni, J. C., Philippakis, A. A., Ellinor, P. T., & Babadi, M. (2023). Unsupervised removal of systematic background noise from droplet-based single-cell experiments using CellBender. Nature Methods , 20(9), 1323–1335. https://doi.org/10.1038/s41592-023-01943-7
- Germain, P.-L., Lun, A., Garcia Meixide, C., Macnair, W., & Robinson, M. D. (2022). Doublet identification in single-cell sequencing data using scDblFinder. F1000Research , 10, 979. https://doi.org/10.12688/f1000research.73600.2
- Germain, P.-L., Sonrel, A., & Robinson, M. D. (2020). pipeComp, a general framework for the evaluation of computational pipelines, reveals performant single-cell RNA-seq preprocessing tools. Genome Biology , 21(1), 227. https://doi.org/10.1186/s13059-020-02136-7
- Grindberg, R. V., Yee-Greenbaum, J. L., McConnell, M. J., Novotny, M., O'Shaughnessy, A. L., Lambert, G. M., Araúzo-Bravo, M. J., Lee, J., Fishman, M., Robbins, G. E., Lin, X., Venepally, P., Badger, J. H., Galbraith, D. W., Gage, F. H., & Lasken, R. S. (2013). RNA-sequencing from single nuclei. Proceedings of the National Academy of Sciences of the United States of America , 110(49), 19802–19807. https://doi.org/10.1073/pnas.1319700110
- Haenni, S., Ji, Z., Hoque, M., Rust, N., Sharpe, H., Eberhard, R., Browne, C., Hengartner, M. O., Mellor, J., Tian, B., & Furger, A. (2012). Analysis of C. elegans intestinal gene expression and polyadenylation by fluorescence-activated nuclei sorting and 3′-end-seq. Nucleic Acids Research , 40(13), 6304–6318. https://doi.org/10.1093/NAR/GKS282
- He, D., Zakeri, M., Sarkar, H., Soneson, C., Srivastava, A., & Patro, R. (2022). Alevin-fry unlocks rapid, accurate and memory-frugal quantification of single-cell RNA-seq data. Nature Methods , 19(3), 316–322. https://doi.org/10.1038/s41592-022-01408-3
- Heiman, M., Kulicke, R., Fenster, R. J., Greengard, P., & Heintz, N. (2014). Cell type–specific mRNA purification by translating ribosome affinity purification (TRAP). Nature Protocols , 9(6), 1282–1291. https://doi.org/10.1038/nprot.2014.085
- Kalish, B. T., Barkat, T. R., Diel, E. E., Zhang, E. J., Greenberg, M. E., & Hensch, T. K. (2020). Single-nucleus RNA sequencing of mouse auditory cortex reveals critical period triggers and brakes. Proceedings of the National Academy of Sciences of the United States of America , 117(21), 11744–11752. https://doi.org/10.1073/pnas.1920433117
- Lacar, B., Linker, S. B., Jaeger, B. N., Krishnaswami, S., Barron, J., Kelder, M., Parylak, S., Paquola, A., Venepally, P., Novotny, M., O'Connor, C., Fitzpatrick, C., Erwin, J., Hsu, J. Y., Husband, D., McConnell, M. J., Lasken, R., & Gage, F. H. (2016). Nuclear RNA-seq of single neurons reveals molecular signatures of activation. Nature Communications , 7, 11022. https://doi.org/10.1038/ncomms11022
- Lähnemann, D., Köster, J., Szczurek, E., McCarthy, D. J., Hicks, S. C., Robinson, M. D., Vallejos, C. A., Campbell, K. R., Beerenwinkel, N., Mahfouz, A., Pinello, L., Skums, P., Stamatakis, A., Attolini, C. S.-O. O., Aparicio, S., Baaijens, J., Balvert, M., Barbanson, B., de, Cappuccio, A., … Schönhuth, A. (2020). Eleven grand challenges in single-cell data science. Genome Biology , 21(1), 31. https://doi.org/10.1186/s13059-020-1926-6
- Lake, B. B., Codeluppi, S., Yung, Y. C., Gao, D., Chun, J., Kharchenko, P. V, Linnarsson, S., & Zhang, K. (2017). A comparative strategy for single-nucleus and single-cell transcriptomes confirms accuracy in predicted cell-type expression from nuclear RNA. Scientific Reports , 7(1), 6031. https://doi.org/10.1038/s41598-017-04426-w
- Lazic, S. E. (2010). The problem of pseudoreplication in neuroscientific studies: is it affecting your analysis? BMC Neuroscience , 11, 5. https://doi.org/10.1186/1471-2202-11-5
- Liddelow, S. A., Marsh, S. E., & Stevens, B. (2020). Microglia and astrocytes in disease: Dynamic duo or partners in crime? Trends in Immunology , 41(9), 820–835. https://doi.org/10.1016/J.IT.2020.07.006
- Lyford, G. L., Yamagata, K., Kaufmann, W. E., Barnes, C. A., Sanders, L. K., Copeland, N. G., Gilbert, D. J., Jenkins, N. A., Lanahan, A. A., & Worley, P. F. (1995). Arc, a growth factor and activity-regulated gene, encodes a novel cytoskeleton-associated protein that is enriched in neuronal dendrites. Neuron , 14(2), 433–445. https://doi.org/10.1016/0896-6273(95)90299-6
- Maitra, M., Nagy, C., Chawla, A., Wang, Y. C., Nascimento, C., Suderman, M., Théroux, J.-F., Mechawar, N., Ragoussis, J., & Turecki, G. (2021). Extraction of nuclei from archived postmortem tissues for single-nucleus sequencing applications. Nature Protocols , 16(6), 2788–2801. https://doi.org/10.1038/s41596-021-00514-4
- Marsh, S. E., Walker, A. J., Kamath, T., Dissing-Olesen, L., Hammond, T. R., de Soysa, T. Y., Young, A. M. H., Murphy, S., Abdulraouf, A., Nadaf, N., Dufort, C., Walker, A. C., Lucca, L. E., Kozareva, V., Vanderburg, C., Hong, S., Bulstrode, H., Hutchinson, P. J., Gaffney, D. J., … Stevens, B. (2022). Dissection of artifactual and confounding glial signatures by single-cell sequencing of mouse and human brain. Nature Neuroscience , 25(3), 306–316. https://doi.org/10.1038/s41593-022-01022-8
- Nguyen, Q. H., Pervolarakis, N., Nee, K., & Kessenbrock, K. (2018). Experimental considerations for single-cell RNA sequencing approaches. Frontiers in Cell and Developmental Biology , 6, 108. https://doi.org/10.3389/fcell.2018.0010
- Nott, A., Schlachetzki, J. C. M., Fixsen, B. R., & Glass, C. K. (2021). Nuclei isolation of multiple brain cell types for omics interrogation. Nature Protocols , 16(3), 1629–1646. https://doi.org/10.1038/s41596-020-00472-3
- Picelli, S., Faridani, O. R., Björklund, Å. K., Winberg, G., Sagasser, S., & Sandberg, R. (2014). Full-length RNA-seq from single cells using smart-seq2. Nature Protocols , 9(1), 171–181. https://doi.org/10.1038/nprot.2014.006
- Rosenberg, A. B., Roco, C. M., Muscat, R. A., Kuchina, A., Sample, P., Yao, Z., Graybuck, L. T., Peeler, D. J., Mukherjee, S., Chen, W., Pun, S. H., Sellers, D. L., Tasic, B., & Seelig, G. (2018). Single-cell profiling of the developing mouse brain and spinal cord with split-pool barcoding. Science , 360(6385), 176–182. https://doi.org/10.1126/science.aam8999
- Squair, J. W., Gautier, M., Kathe, C., Anderson, M. A., James, N. D., Hutson, T. H., Hudelle, R., Qaiser, T., Matson, K. J. E., Barraud, Q., Levine, A. J., la Manno, G., Skinnider, M. A., & Courtine, G. (2021). Confronting false discoveries in single-cell differential expression. Nature Communications , 12(1), 5692. https://doi.org/10.1038/s41467-021-25960-2
- Stegle, O., Teichmann, S. A., & Marioni, J. C. (2015). Computational and analytical challenges in single-cell transcriptomics. Nature Reviews Genetics , 16(3), 133–145. https://doi.org/10.1038/nrg3833
- Steward, O., Wallace, C. S., Lyford, G. L., & Worley, P. F. (1998). Synaptic activation causes the mRNA for the IEG Arc to localize selectively near activated postsynaptic sites on dendrites. Neuron , 21(4), 741–751. https://doi.org/10.1016/S0896-6273(00)80591-7
- Stuart, T., Butler, A., Hoffman, P., Stoeckius, M., Smibert, P., Satija, R., Stuart, T., Butler, A., Hoffman, P., Hafemeister, C., Papalexi, E., Iii, W. M. M., Hao, Y., Stoeckius, M., Smibert, P., & Satija, R. (2019). Comprehensive integration of single-cell data resource comprehensive integration of single-cell data. Cell , 177(7), 1888–1902.e21. https://doi.org/10.1016/j.cell.2019.05.031
- Svensson, V., Vento-Tormo, R., & Teichmann, S. A. (2018). Exponential scaling of single-cell RNA-seq in the past decade. Nature Protocols , 13(4), 599–604. https://doi.org/10.1038/nprot.2017.149
- Tang, F., Barbacioru, C., Wang, Y., Nordman, E., Lee, C., Xu, N., Wang, X., Bodeau, J., Tuch, B. B., Siddiqui, A., Lao, K., Surani, A., & Surani, M. A. (2009). mRNA-seq whole-transcriptome analysis of a single cell. Nature Methods , 6(5), 377–382. https://doi.org/10.1038/nmeth.1315
- van den Brink, S. C., Sage, F., Vértesy, Á., Spanjaard, B., Peterson-Maduro, J., Baron, C. S., Robin, C., & van Oudenaarden, A. (2017). Single-cell sequencing reveals dissociation-induced gene expression in tissue subpopulations. Nature Methods , 14(10), 935–936. https://doi.org/10.1038/nmeth.4437
- von Ziegler, L. M., Floriou-Servou, A., Waag, R., Das Gupta, R. R., Sturman, O., Gapp, K., Maat, C. A., Kockmann, T., Lin, H. Y., Duss, S. N., Privitera, M., Hinte, L., von Meyenn, F., Zeilhofer, H. U., Germain, P. L., & Bohacek, J. (2022). Multiomic profiling of the acute stress response in the mouse hippocampus. Nature Communications , 13(1), 1824. https://doi.org/10.1038/s41467-022-29367-5
- Wang, X., He, Y., Zhang, Q., Ren, X., & Zhang, Z. (2021). Direct comparative analyses of 10x genomics chromium and Smart-seq2. Genomics, Proteomics & Bioinformatics, 19(2), 253–266. https://doi.org/10.1016/J.GPB.2020.02.005
- Wu, H., Kirita, Y., Donnelly, E. L., & Humphreys, B. D. (2019). Advantages of single-nucleus over single-cell RNA sequencing of adult kidney: rare cell types and novel cell states revealed in fibrosis. Journal of the American Society of Nephrology , 30(1), 23–32. https://journals.lww.com/jasn/fulltext/2019/01000/advantages_of_single_nucleus_over_single_cell_rna.7.aspx
- Wu, Y. E., Pan, L., Zuo, Y., Li, X., & Hong, W. (2017). Detecting activated cell populations using single-cell RNA-seq. Neuron , 96(2), 313–329.e6. https://doi.org/10.1016/j.neuron.2017.09.026
- Zeisel, A., Muñoz-Manchado, A. B., Codeluppi, S., Lönnerberg, P., la Manno, G., Juréus, A., Marques, S., Munguba, H., He, L., Betsholtz, C., Rolny, C., Castelo-Branco, G., Hjerling-Leffler, J., & Linnarsson, S. (2015). Cell types in the mouse cortex and hippocampus revealed by single-cell RNA-seq. Science , 347(6226), 1138–1142. https://doi.org/10.1126/science.aaa1934
- Zheng, G. X. Y., Terry, J. M., Belgrader, P., Ryvkin, P., Bent, Z. W., Wilson, R., Ziraldo, S. B., Wheeler, T. D., McDermott, G. P., Zhu, J., Gregory, M. T., Shuga, J., Montesclaros, L., Underwood, J. G., Masquelier, D. A., Nishimura, S. Y., Schnall-Levin, M., Wyatt, P. W., Hindson, C. M., … Bielas, J. H. (2017). Massively parallel digital transcriptional profiling of single cells. Nature Communications , 8, 14049. https://doi.org/10.1038/ncomms14049
Citing Literature
Number of times cited according to CrossRef: 1
- Anke M. Tukker, Aaron B. Bowman, Application of single cell gene expression technologies to neurotoxicology, Current Opinion in Toxicology, 10.1016/j.cotox.2023.100458, 37 , (100458), (2024).