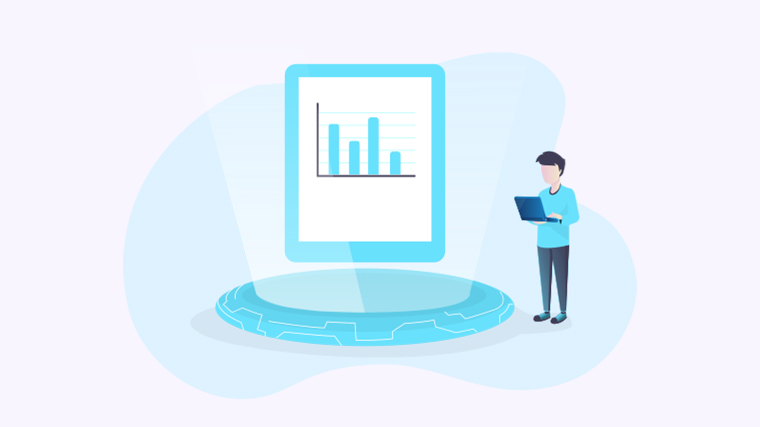
比较了11种RNA-seq数据的差异表达分析方法。主要结果如下:
DESeq - Conservative with default settings. Becomes more conservative when outliers are introduced.
- Generally low TPR.
- Poor FDR control with 2 samples/condition, good FDR control for larger sample sizes, also with outliers.
- Medium computational time requirement, increases slightly with sample size.
edgeR - Slightly liberal for small sample sizes with default settings. Becomes more liberal when outliers are introduced.
- Generally high TPR.
- Poor FDR control in many cases, worse with outliers.
- Medium computational time requirement, largely independent of sample size.
NBPSeq - Liberal for all sample sizes. Becomes more liberal when outliers are introduced.
- Medium TPR.
- Poor FDR control, worse with outliers. Often truly non-DE genes are among those with smallest p-
values.
- Medium computational time requirement, increases slightly with sample size.
TSPM - Overall highly sample-size dependent performance.
- Liberal for small sample sizes, largely unaffected by outliers.
- Very poor FDR control for small sample sizes, improves rapidly with increasing sample size.
Largely unaffected by outliers.
- When all genes are overdispersed, many truly non-DE genes are among the ones with smallest p-
values. Remedied when the counts for some genes are Poisson distributed.
- Medium computational time requirement, largely independent of sample size.
voom / vst
- Good type I error control, becomes more conservative when outliers are introduced.
- Low power for small sample sizes. Medium TPR for larger sample sizes.
- Good FDR control except for simulation study B04000. Largely unaffected by introduction of outliers.
- Computationally fast.
baySeq - Highly variable results when all DE genes are regulated in the same direction. Less variability when the DE genes are regulated in different directions.
- Low TPR. Largely unaffected by outliers.
- Poor FDR control with 2 samples/condition, good for larger sample sizes in the absence of outliers. Poor FDR control in the presence of outliers.
- Computationally slow, but allows parallelization.
EBSeq - TPR relatively independent of sample size and presence of outliers.
- Poor FDR control in most situations, relatively unaffected by outliers.
- Medium computational time requirement, increases slightly with sample size.
NOISeq - Not clear how to set the threshold for qNOISeq to correspond to a given FDR threshold.
- Performs well, in terms of false discovery curves, when the dispersion is different between the
conditions (see supplementary material).
- Computational time requirement highly dependent on sample size.
SAMseq - Low power for small sample sizes. High TPR for large enough sample sizes.
- Performs well also for simulation study B04000.
- Largely unaffected by introduction of outliers.
- Computational time requirement highly dependent on sample size.
ShrinkSeq - Often poor FDR control, but allows the user to use also a fold change threshold in the inference procedure.
- High TPR.
- Computationally slow, but allows parallelization.
没有哪种单独的方法对所有情形都是最优的,特定情形下方法的选择取决于实验条件。本文评价的这些方法中,基于稳定方差的变换与limma组合的方法在很多情况下都表现不错,而且不受例外点影响、计算很快,但是要求每条件下至少3个样本来提供充分的检定力。而且在两条件下散度不同时表现更糟糕。非参数方法SAMseq在大样本量时是性能最优的方法,需要至少每条件下4-5个样本提供充分的检定力。对于高表达基因,SAMseq的统计显著性所需的倍数变化比很多其他方法要低,这可能潜在地折中了一些统计显著的DEGs的生物学显著性。对ShrinkSeq也是一样,不过它有一个选项在推断过程中强加一个倍数变化要求。
小样本导致一些方法的误报率远超FDR阈值。对于参数方法,这可能是因为均值和方差估计不精确。TSPM受样本量影响最大,可能因为它使用了渐进估计。尽管发展指向大样本量,而且barcoding和multiplexing创造了固定成本分析更多样本的机会,但是目前为止RNA-seq实验仍然太贵而不允许广泛的重复。本研究所传达的结果强烈建议小样本差异表达基因应该谨慎解释,真实FDR可能超出所选FDR阈值数倍。
DESeq、edgeR和NBPSeq基于类似的原理,因此基因排序的精确度很类似。但是相同阈值选取出的DEGs有很大不同,这是因为它们估计散度参数的方法不同。在缺省设置和合理的大样本量下,DESeq通常过于保守而edgeR和NBPSeq通常过于慷慨而得出大量假DEGs。分析表明参数选择影响很大,而且缺省推荐参数事实上选择的很好通常能得到最佳结果。
EBSeq、baySeq、ShrinkSeq使用了不同的推断方法来估计每个基因差异表达的后验概率。baySeq一些条件下表现不错,但是高度可变,特别是所有基因都上调或都下调时。大样本量条件下有异常值时,EBSeq比baySeq的假阳性低,小样本量时baySeq比EBSeq的假阳性低。
原文:http://blog.sina.com.cn/s/blog_3eaf29360101n5lv.html
欢迎关注